20 Standout RAG Use Cases That Deliver Real Value with GenAI
Published on Jun 19, 2025
Get Started
Fast, scalable, pay-per-token APIs for the top frontier models like DeepSeek V3 and Llama 3.3. Fully OpenAI-compatible. Set up in minutes. Scale forever.
Have you ever been frustrated with the limited capabilities of a chatbot or virtual assistant? Perhaps they couldn't comprehend your request or provide a satisfactory answer. What if you could improve their performance and accuracy by grounding their responses in a wealth of trusted information? That's the promise of RAG, which is short for retrieval-augmented generation. RAG combines the strengths of generative models and retrieval systems to produce accurate, context-aware responses. Built on advanced Machine Learning Frameworks, this article will cover RAG use cases and examples to illustrate how to build smarter, faster, and more reliable AI systems that deliver real-world value by grounding generative models in trusted, up-to-date information.
One effective way to achieve your goals around RAG is to leverage Inference's AI inference APIs. With our solution, you can reduce the time and cost of development, and get to the business of building accurate, reliable AI systems faster.
What is Retrieval-Augmented Generation (RAG)?
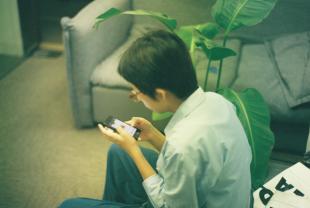
Retrieval-augmented generation, or RAG, is a method that combines retrieval of external documents with generative AI to produce more accurate and informed responses. RAG enhances large language models by grounding their output in up-to-date or domain-specific information.
Here’s how it works:
RAG first retrieves relevant data for a prompt or question, then feeds that information as context to a generative AI model like a large language model before generating a response. This approach can significantly improve the accuracy of LLM applications, especially in areas like customer support chatbots and Q&A systems that need to maintain up-to-date information or access domain-specific knowledge.
What Challenges Does Retrieval-Augmented Generation (RAG) Solve?
Retrieval-augmented generation can improve the efficacy of large language model (LLM) applications by leveraging custom data. This is done by retrieving data/documents relevant to a question or task and providing them as context for the LLM. RAG has successfully supported chatbots and Q&A systems that need to maintain up-to-date information or access domain-specific knowledge.
Problem 1
- LLM models do not know your data
- LLMs use deep learning models and train on massive datasets to understand, summarize, and generate novel content.
Most LLMs are trained on a wide range of public data, so one model can respond to many tasks or questions. Once trained, many LLMs cannot access data beyond their training data cutoff point.
This makes LLMs static and may cause them to respond incorrectly, give out-of-date answers, or hallucinate when asked questions about data they have not been trained on.
Problem 2
AI applications must leverage custom data to be effective. For LLMs to give relevant and specific responses, organizations need the model to understand their domain and provide answers from their data, rather than offering broad and generalized responses.
Customization Without Retraining
For example, organizations build customer support bots with LLMs, and those solutions must give company-specific answers to customer questions. Others are building internal Q&A bots that should answer employees' questions on internal HR data. How do companies make such solutions without retraining those models?
How a RAG Application Works
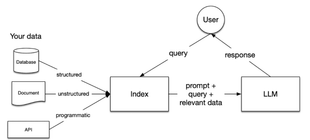
What Happens Inside a RAG Application?
RAG, or retrieval-augmented generation, combines the strengths of large language models (LLMs) and traditional information retrieval techniques. The "retrieval" component enables LLMs to find accurate information from external sources before generating a response to a user query.
Understanding the RAG Application Flow
Here’s what happens inside a typical rag application: User → Question → Retriever → External Data → LLM (Generator) → Final Response. Let’s break it down: The user asks a question. A retriever searches internal or external content (PDFs, databases, websites). The most relevant piece of data is pulled in.
That content is passed into the large language model (LLM). The model uses it to generate a fact-aware response. That’s how the best rag examples deliver sharp, grounded answers—without training or manual tuning.
Why RAG Beats Fine-Tuning and Prompt Engineering
Prompt engineering is hit or miss. Fine-tuning requires time, money, and a substantial amount of labeled data. RAG skips both. It provides your AI model with real-time access to accurate information—no need to bake everything into the model.
No need to guess what prompt trick will work. If your product, chatbot, or assistant depends on fast, factual answers, a RAG use case is your most reliable play. RAG is quicker to build, easier to maintain, and better at scaling across topics or use cases.
Why Use Retrieval-Augmented Generation (RAG)?
RAG solves several critical problems that traditional AI and knowledge management systems face, such as:
- Hallucination RAG ensures that responses are based on actual documents and data rather than fabricated or mixed-up information. This grounding in real sources allows for accurate and reliable answers.
- Knowledge freshness Implementing RAG allows users to access and use up-to-date information, eliminating the limitations of outdated training data. This means organizations can incorporate new information instantly, ensuring that AI responses reflect the most current data.
- Private or proprietary knowledge access RAG can handle company-specific information, allowing it to work seamlessly with internal documents, policies, and procedures. This enables organizations to answer questions related to their unique practices and guidelines.
- Contextual accuracy. With RAG, responses are tailored based on the documents relevant to the inquiry. This ensures that answers are specific, rather than generic, leading to more valuable and actionable information.
- Knowledge discovery RAG facilitates quick and relevant information retrieval, making it easy to locate specific details within large document sets. This enhances efficiency by allowing users to find important information rapidly, even in extensive databases.
Related Reading
20 Innovative RAG Use Cases for Faster, Context-Aware AI Output
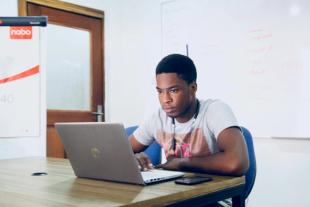
1. Retrieval-augmented generation, or RAG
RAG enhances customer support chatbots by combining retrieval-based systems with generative AI to provide accurate and contextually relevant responses. When a customer asks a question, the chatbot retrieves relevant information from sources like:
- Knowledge bases
- FAQs
- Customer records
Retrieval-Augmented Generation (RAG)
It uses a generative model to craft a personalized response based on the retrieved data. This enables chatbots to handle complex queries that require up-to-date, detailed information.
For example, Shopify’s Sidekick chatbot—designed to automatically ingest Shopify store data—leverages retrieval-augmented generation (RAG) to deliver superior AI customer service by offering precise answers related to:
- Products
- Account issues
- Troubleshooting
Sidekick enhances the e-commerce experience by pulling relevant data from:
- Store inventories
- Order histories
- FAQs
This helps provide dynamic, contextually accurate responses in real time. Similarly, Google Cloud's Contact Center AI integrates RAG to offer personalized, real-time solutions, assisting customers to resolve issues faster while reducing the need for human agents.
2. Document Summarization and Search: Easy Peasy
Retrieval-augmented generation (RAG) has emerged as an efficient document summarization and search technology. It leverages advanced information retrieval techniques to enhance the capabilities of large language models (LLMs).
Efficient Retrieval with RAG
RAG systems can provide efficient results by integrating retrieval methods such as approximate nearest neighbor (ANN) algorithms with complex ranking models. For example, Google's Vertex AI Search uses a two-stage retrieval process: It first uses approximate nearest neighbor (ANN) algorithms to gather potential results quickly, then applies deep learning models for re-ranking to ensure the most relevant documents are prioritized.
This approach enhances the accuracy of search results. It allows for extracting critical information from documents, ensuring that users receive concise and contextually relevant answers without the noise of irrelevant content.
RAG in Financial Analysis
In finance, Bloomberg has implemented RAG to streamline summarization of extensive financial documents, like earnings reports, by pulling the latest data and extracting insights. This system improves analysts' decision-making by providing real-time summaries tailored to current financial contexts.
Up-to-date information is critical in fast-moving environments. It enhances the relevance of summaries provided to users and supports their strategic decisions.
3. Medical Diagnostics and Research: Making Sense of the Data
RAG (retrieval-augmented generation) marks a notable medical diagnostics and research advancement. RAG systems utilize vast medical knowledge databases, including electronic health records, clinical guidelines, and medical literature, to support healthcare professionals in making accurate diagnoses and well-informed treatment decisions.
RAG in Medical Diagnostics
Tools like IBM Watson Health exemplify this application. Utilizing natural language processing and machine learning algorithms, IBM Watson analyzes patient data against extensive medical literature, aiding doctors in diagnosing complex cases more effectively. This platform helps oncologists determine personalized treatment options based on a patient's:
- Unique genetic profile
- Latest research findings
RAG for Cancer Treatment
One notable application is IBM Watson Health, which employs RAG techniques to analyze large datasets, including electronic health records (EHRs) and medical literature, to aid in cancer diagnosis and treatment recommendations.
Watson’s ability to retrieve relevant clinical studies and generate personalized treatment plans based on individual patient profiles illustrates how RAG can optimize decision-making in healthcare settings.
RAG's Accuracy in Oncology
According to a study published in the Journal of Clinical Oncology, IBM Watson for Oncology matched treatment recommendations with expert oncologists 96% of the time, showcasing the potential of RAG to augment human expertise in medical diagnostics. Integrating such technology:
- Enhances patient outcomes
- Reduces the cognitive load on healthcare professionals.
This allows them to focus on patient care rather than data management.
4. Personalized Learning and Tutoring Systems: Your Academic Assistant
When it comes to personalized learning, RAG combines the power of large language models (LLMs) with retrieval systems to offer students more relevant and precise guidance. A notable example is RAMO (retrieval-augmented generation for MOOCs), which uses LLMs to generate personalized course suggestions and address the "cold start" problem in course recommendations.
Personalized E-Learning with RAG
Through conversational interfaces, RAMO assists learners by understanding their preferences and career goals, offering more relevant course options, and enhancing the e-learning experience. Beyond course recommendations, RAG-powered systems are used in intelligent tutoring in higher education.
Intelligent Tutoring with LLMs
LLMs, integrated with retrieval mechanisms, help create intelligent agent tutors that deliver personalized instruction and real-time student feedback. These systems adapt to individual learning paths by retrieving relevant knowledge and combining it with generated explanations to guide learners through complex topics.
For example, universities have begun deploying RAG-driven tutoring systems to assist students in navigating course materials more effectively, fostering more profound understanding and improving academic outcomes.
5. Fraud Detection and Risk Assessment: Finding the Bad Guys
Companies implementing RAG have reported significantly improved fraud detection rates compared to traditional machine learning models. This is primarily due to RAG's ability to access and incorporate real-time, relevant data during decision-making. Conventional methods rely heavily on pre-defined rules and historical data, which can be limited in scope and may miss emerging fraud patterns.
RAG for Dynamic Fraud Detection
RAG, enables dynamic, contextual data retrieval, enhancing the system’s ability to detect anomalies by integrating up-to-date, external information like newly reported fraud schemes or regulatory changes. Financial companies like JPMorgan Chase use AI-driven fraud detection systems using retrieval-augmented generation RAG models.
Real-Time Fraud Analysis Systems
These systems continuously retrieve and analyze real-time data from various sources to monitor transactions and detect potential fraud. Similar to RAG, they combine data retrieval with advanced analytics to assess transactions in context, enhancing the accuracy and responsiveness of fraud detection.
In retail banking, these systems can cross-reference transaction data with external fraud reports and blocklists to flag suspicious activities with greater precision, reducing the number of false positives that typically overwhelm traditional rule-based approaches.
6. E-commerce Product Recommendations: Finding the Right Products
Retrieval-augmented generation (RAG) is revolutionizing e-commerce product recommendations by combining generative AI and retrieval systems to provide highly personalized shopping experiences.
RAG models first retrieve the relevant product information from external knowledge bases or a company's product catalogue and then generate recommendations based on the user's preferences, search behaviour, and historical data.
Dynamic Recommendations with RAG
Unlike traditional recommendation systems that rely solely on predefined algorithms or collaborative filtering, RAG dynamically tailors suggestions by understanding specific customer needs in real-time. This results in more relevant and accurate recommendations, boosting user engagement and increasing sales.
Amazon's RAG-Enhanced Product Suggestions
For example, Amazon has integrated AI-driven recommendation engines that utilize retrieval-augmented generation (RAG) techniques to enhance e-commerce product recommendations.
The COSMO framework leverages large language models (LLMs) alongside a knowledge graph capturing commonsense relationships from customer behavior, enabling the system to generate contextually relevant suggestions.
Zalando's Personalized Fashion Recommendations
Similarly, Zalando has been experimenting with RAG models to suggest fashion items based on users' past interactions and preferences, significantly improving the shopping experience. These real-world applications of RAG showcase its potential to transform how e-commerce platforms deliver personalized shopping experiences.
7. Enterprise Knowledge Management: Getting Answers Fast
RAG models combine generative AI with retrieval mechanisms, allowing enterprises to generate contextually accurate responses by pulling relevant information from their proprietary knowledge bases. This is particularly beneficial for large companies with extensive documentation and data sources.
RAG for Efficient Information Retrieval
Using RAG, enterprises can provide employees and customers with instant, tailored answers to queries, reducing the need for manual search and improving efficiency. Siemens utilizes retrieval-augmented generation (RAG) technology to enhance internal knowledge management. Integrating RAG into its digital assistance platform lets employees quickly retrieve information from various internal documents and databases.
Siemens' RAG-Powered Knowledge Management
Users can input queries when faced with technical questions, and the RAG model provides relevant documents and contextual summaries. This approach improves response times and fosters collaboration, ensuring all employees have access to up-to-date information, ultimately driving innovation and reducing redundancy.
Morgan Stanley's RAG for Wealth Management
Another notable application is at Morgan Stanley, which uses retrieval-augmented generation (RAG) technology in its Wealth Management division to enhance its internal knowledge management and improve the efficiency of its financial advisors. The firm has partnered with OpenAI to create a bespoke solution that enables financial advisors to access and synthesize various internal insights related to quickly:
- Companies
- Sectors
- Market trends
This system retrieves data and generates explanatory text, ensuring that advisors receive precise answers to complex queries.
8. Delivery Support Chatbot: Keeping Delivery Drivers on Track
Doordash, a food delivery company, enhances delivery support with a RAG-based chatbot. The company developed an in-house solution that combines three key components: the RAG system, the LLM guardrail, and the LLM judge. When a “Dasher,” an independent contractor who does deliveries through DoorDash, reports a problem, the system first condenses the conversation to grasp the core issue accurately.
RAG-Powered Customer Support
Using this summary, it then searches the knowledge base for the most relevant articles and past resolved cases. The retrieved information is fed into an LLM, which crafts a coherent and contextually appropriate response tailored to Dasher's query. To maintain the high quality of the system’s responses, DoorDash implemented the LLM Guardrail system, an online monitoring tool that evaluates each LLM-generated response for accuracy and compliance.
Quality Control and Monitoring
It helps prevent hallucinations and filter out responses that violate company policies. To monitor the system quality over time, DoorDash uses an LLM Judge that assesses the chatbot's performance across five LLM evaluation metrics: retrieval correctness, response accuracy, grammar and language accuracy, coherence to context, and relevance to the Dasher's request.
9. AI Professor: Your New Academic Assistant
Harvard Business School's Senior Lecturer, Jeffrey Bussgang, created a RAG-based AI faculty chatbot to help him teach his entrepreneurship course. The chatbot, ChatLTV, helps students with course preparation, like:
- Clarifying complex concepts
- Finding additional information on case studies
- Administrative matters
ChatLTV's Training and Integration
ChatLTV was trained on the course corpus, including case studies, teaching notes, books, blog posts, and historical Q&A from the course's Slack channel. The chatbot is integrated into the course's Slack channel, allowing students to interact with it in private and public modes. The LLM is provided with the query and relevant context stored in a vector database to respond to a student's question.
Response Accuracy and Testing
The most relevant content chunks are served to the LLM using OpenAI's API. To ensure ChatLTV’s responses are accurate, the course team used a mix of manual and automated testing. They used an LLM judge to compare the outputs to the ground-truth data and generate a quality score.
10. Research and Content Creation: Finding Reliable Sources Fast
Writers, journalists, and researchers can use RAG to pull accurate references from trusted sources, simplifying and speeding up the fact-checking process and information gathering. Whether drafting an article or compiling data for a study, RAG makes it easy to quickly access relevant and credible material, allowing creators to focus on producing high-quality content.
11. Educational Tools: Personalized Learning Tools
RAG can be used in educational platforms to provide students with detailed explanations and contextually relevant examples, drawing from various educational materials. Duolingo uses RAG for personalized language instruction and feedback, while Quizlet employs it to generate tailored practice questions and provide user-specific feedback.
12. Decision Support Systems: Making Decisions Easier
Managers and decision-makers often need access to up-to-date information from various sources to make informed decisions. RAGs can provide a consolidated view by retrieving data from multiple channels, summarizing it, and presenting actionable insights. This reduces the time spent on research and offers a holistic view of strategic decisions.
Example Use Case
A financial analyst could use a RAG-powered system to pull data from market trends, competitor reports, and internal financials and generate a report that assists the company’s executive team make strategic investment decisions.
13. Research and Development (R&D): Accelerating Progress
For companies in R&D-heavy sectors like pharmaceuticals, technology, or engineering, RAGs can assist in retrieving research papers, patents, technical documentation, and more. Instead of manually combing through countless papers and documents, RAGs can retrieve and summarize key findings.
RAG for Research Acceleration
This would accelerate the research process and allow researchers to focus on innovation. They can also generate insights from these sources, helping researchers stay up-to-date with the latest developments in their field. Moreover, since RAGs can integrate information from various fields, they can assist in finding novel insights that may not be apparent from a single discipline.
Example Use Case
A pharmaceutical company could use a RAG-powered tool to scan the latest medical research on a particular compound. The tool can pull out key findings and generate a report highlighting the potential benefits and risks for further investigation.
14. Customer Support Assistants: Smarter Chatbots for Better Customer Support
Customer support assistants are an application designed to power more innovative customer support chatbots. Instead of relying on pre-written replies or guessing, the bot pulls answers directly from your real documents, like:
- Help center articles
- User guides
- Past support tickets
It uses that live info to respond more accurately and clearly to each question.
Why RAG is Useful Here
Most chatbots today sound generic or simply don’t assist. They can’t access your latest content, and they struggle with anything outside their scripts. That leads to frustrated users and extra work for your support team. RAG fixes that by connecting your chatbot to your actual knowledge base. It helps the bot respond with facts—not guesses—so users receive helpful answers more quickly.
It also means your team handles fewer repetitive tickets. This is one of the most effective RAG use cases in the real world.
Tools or Tech Stack for the Nerds Vector Store
- Pinecone
- Weaviate
Elasticsearch LLM: OpenAI GPT-4, Claude, or Cohere Framework: LangChain or LlamaIndex Support system integrations: Botpenguin UI: Web chat, live chat widget, WhatsApp bot.
15. AI-Powered Search Engines: More Than Just a Pretty Interface
This RAG application turns a simple search bar into something way more innovative. Instead of showing a list of links, it retrieves the most relevant information and generates a direct, helpful answer. Whether it’s for a product catalog, a documentation site, or a media archive, RAG adds intelligence where keyword search fails. It doesn’t just look for words. It understands meaning.
Why RAG is Useful Here
Most search engines struggle with how people ask questions. They rely on:
- Exact matches
- Outdated indexing
- Confusing filters
The Solution to Information Overload
Users bounce because they can’t find what they need fast enough. RAG solves this by blending search and generation. It pulls context-aware content from your database or content library, then uses a language model to create a clear, natural response. That’s what makes this RAG use case so powerful, especially for companies dealing with:
- Large
- Messy
- Unstructured data
Tools or Tech Stack for the Nerds
Content Sources
- Website content
- Product catalogs
- Research databases
Vector DBs
- Pinecone
- Vespa
- Weaviate
Large Language Models (LLMs)
- OpenAI
- Claude
- Mistral
Frameworks
- LangChain
- LlamaIndex
Frontend
- React
- Next.js
- Chatbot wrapper (if conversational)
User Interface (UI)
- Search bar interface
- Chatbot overlay
- Autocomplete dropdown
16. Legal Document Analysis: The Application That Gets Lawyers
This RAG application enables legal professionals to search, review, and summarize complex legal documents efficiently, eliminating the need to spend hours sifting through PDFs or databases.
It retrieves relevant clauses, cases, or regulations and uses an LLM to explain them clearly, right inside a chat or search tool. Think of it as a legal assistant that never misses a detail and doesn’t bill by the hour.
Why RAG is Useful Here
Legal teams deal with vast volumes of dense, repetitive text. Traditional search tools fall short. They can’t understand intent or nuance, and they often miss key context. RAG changes that. By connecting to your internal contracts, regulatory libraries, or case archives, it retrieves exactly what matters—and breaks it down in plain language.
This RAG use case helps law firms, compliance teams, and in-house legal ops:
- Save time
- Reduce risk
- Work smarter
No more Ctrl+F. No more digging through folders. Just answers.
Tools or Tech Stack for the Nerds: Document Sources
- Contract databases
- SEC filings
- GDPR/CCPA docs
Vector DBs
- Qdrant
- Vespa
- Milvus
Large Language Models (LLMs)
- GPT-4
- Claude 2
- LegalBERT
Frameworks
- LangChain
- Haystack
- LlamaIndex
Compliance Layer
- Role-based access
- Audit logging
- Redaction filters
User Interface (UI)
- Legal dashboard
- Contract explorer
- Sidebar chat in DMS
7. Education and Tutoring Platforms: Tailored Learning at Scale
This RAG application powers intelligent tutoring assistants that provide students with fast, accurate help across subjects, drawn directly from trusted educational materials. Instead of copying generic answers from the internet, the AI retrieves content from:
- Approved textbooks
- Lesson plans
- Class notes
Then it explains it clearly in the student’s language. It’s like having a personal tutor who knows your syllabus.
Why RAG is Useful Here
Every student learns differently. Most search tools or AI tutors provide broad or oversimplified responses, often pulling information from unreliable sources. RAG changes that. It connects to course-specific content, worksheets, or instructor notes and delivers:
- Custom explanations
- Step-by-step breakdowns
- Real examples
This makes it one of the most impactful RAG use cases in edtech, enabling learners to delve deeper, faster, and with fewer distractions. Teachers save time. Students gain confidence. Learning becomes more personal.
Tools / Tech Stack for the Nerds
Data Sources
- PDFs
- E-learning modules
- LMS exports
- Lecture transcripts
Retrieval
- Pinecone
- Weaviate
- Vespa
Large Language Models (LLMs)
- GPT-4
- Claude
- Mistral
Frameworks
- LangChain
- LlamaIndex
- Haystack
Delivery
- Chat-based UI
- Web widgets
- Mobile apps
User Interface (UI)
- In-app tutor chat
- Lesson chatbot
- LMS widget
8. Codebase Navigation Tools: The Search Bar on Steroids
This RAG application enables developers to ask natural questions about large, complex codebases and receive precise answers instantly. Instead of digging through dozens of files or Stack Overflow threads, they can simply ask:
- What does this function do?
- Where is the auth logic handled?
- What are the dependencies of this module?
The system retrieves code chunks, context, and explanations using the RAG method.
Why RAG is Useful Here
Big codebases slow everyone down. New devs struggle to onboard. Seniors waste time answering the same questions. Docs are outdated or missing entirely. That’s where RAG wins. It connects your AI assistant to your live codebase, commits, and documentation. When a developer requests information, the system retrieves the relevant snippet and explains it in plain language.
This RAG use case reduces cognitive load, speeds up onboarding, and gives engineers more time to build.
Tools / Tech Stack for the Nerds
Data Sources
- Git repos
- Markdown docs
- Code comments
READMEs Retrieval
- Qdrant
- Weaviate
- Elasticsearch
Large Language Models (LLMs)
- GPT-4
- Claude 2
- CodeLlama
Frameworks
- LlamaIndex
- LangChain
- Graph
RAG (for file trees) UI
- IDE chat (VSCode)
- Developer console
- Git UI overlay
19. News and Research Summarizers: The Personal Assistant You Didn’t Know You Needed
This rag application helps users cut through information overload by summarizing news, research papers, and reports into digestible insights. Instead of relying on static summaries or generic recaps, it pulls content from trusted sources in real-time, then turns it into fast, focused, and human-readable answers. It’s like having a personal analyst, researcher, and editor in one.
Why RAG is Useful Here
People don’t have time to read everything. And most summaries miss context or skip what matters. That’s the gap. With RAG, the assistant retrieves the most relevant paragraphs, quotes, or charts, then explains the highlights clearly. It adapts based on what the user asks, not just what’s in the feed.
This rag use case is especially valuable for journalists, researchers, executives, and knowledge workers who want to stay current without getting overwhelmed by tabs. Less skimming. More knowing.
Tools / Tech Stack for the Nerds
Content Sources
- RSS feeds
- Academic journals
- Press releases
- Company blogs
Vector Search
- Pinecone
- Vespa
- Qdrant
Large Language Models (LLMs)
- GPT-4
- Claude
- Mistral
Frameworks
- LangChain
- LlamaIndex
Delivery
- Chat interface
- Slack digest
- Email summaries
- Browser extension
User Interface (UI)
- Research dashboard
- Email bot
- Slack app
20. Conversational Agents for CRM or ERP Systems: Smarter Business Software
This RAG application integrates with your CRM or ERP system and powers a chatbot that can answer business-critical questions. Instead of navigating through endless dashboards and filters, users can ask:
- What’s the status of the Acme Corp invoice?
- Who’s our top-performing sales rep this quarter?
- Did we close that deal in Q2?
The assistant fetches and explains the answer instantly, right inside the chat.
Why RAG is Useful Here
CRMs and ERPs are packed with data, but they struggle to help humans effectively utilize it. You have to know where to click, what to filter, or which tab hides the number you're looking for. That’s not just annoying; it slows decisions. RAG removes that friction. It understands the question, pulls from structured records and unstructured notes, and generates a clear answer. Fast.
This RAG use case is a game changer for sales teams, account managers, finance ops, and support, where fast answers create real results.
Tools / Tech Stack for the Nerds
Data Sources
- Salesforce
- HubSpot
- Zoho
- SAP
Oracle Retrieval
- Weaviate
- Elasticsearch
- Qdrant
Large Language Models (LLMs)
- GPT-4
- Claude 2
- Mistral
Frameworks
- LangChain
- LlamaIndex
- Private API connectors
User Interface (UI)
- Slackbots
- Microsoft Teams integrations
- CRM sidebar widgets
Related Reading
How to Choose the Right RAG Use Case for Your Needs
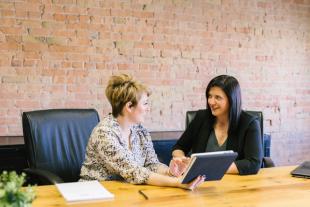
Not every RAG solution fits every business. Here’s how to choose the RAG application that solves your problem, without wasting time or budget:
Start With the Pain Point, Not the Technology
Before selecting any RAG use case, clarify the core frustration you're solving. Is your team overwhelmed by support tickets? Are your sales reps struggling to find CRM data? Is knowledge trapped across folders no one reads? RAG works best when there is a clear signal, such as high volume, repetitive questions, or slow access to information. That's your cue.
Match RAG Strengths to Your Workflow
A good RAG application isn't just cool tech; it fits where your users already are. If your users work in Slack or Teams, build there. If they search internal docs, connect the RAG layer to those sources. If you're customer-facing, plug it into your chatbot or site search. Use RAG to improve workflows, not add new ones.
Consider Your Data Type and Format
RAG thrives on unstructured content:
- PDFs
- Docs
- Transcripts
- Wikis
Nevertheless, not all RAG use cases require the same data preparation. Product search needs structured specs. Legal tools need contracts. Internal assistants need help, docs, and policies. Think about where your "answers" live, and whether they're ready to be retrieved.
Pick Use Cases With High Return on Clarity
The best RAG applications don't just automate; they reduce confusion. Use cases like:
- Customer support chatbots
- Internal knowledge search
- Sales enablement in CRM
- Research summarizers
These drive instant value by turning complexity into clarity. If it replaces a meeting, email, or long search, it's a strong candidate.
Align With Tools You Already Use
Look at your current stack: CRM, ERP, LMS, Helpdesk, Search. Many RAG examples start by connecting to tools teams already use, then layering a conversational interface or better retrieval logic. No need to rip and replace. Start by enhancing what already exists.
Start Small. Prove Fast.
You don't need to deploy across your org on day one. The most successful RAG applications begin small, addressing one painful, high-impact problem within a single department. Prove it. Scale it. Then expand. Need inspiration? Scroll back up to the 20 real-world RAG use cases above—each one is a blueprint waiting to be tailored to your needs.
Start Building with $10 in Free API Credits Today!
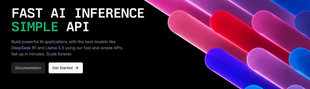
Inference delivers OpenAI-compatible serverless inference APIs for top open-source LLM models, offering developers the highest performance at the lowest cost in the market. Beyond standard inference, Inference provides specialized batch processing for large-scale async AI workloads and document extraction capabilities designed explicitly for RAG applications.
Start building with $10 in free API credits and experience state-of-the-art language models that balance cost-efficiency with high performance.