What Is Feature Scaling in Machine Learning & When You Should Use It?
Published on Apr 20, 2025
Get Started
Fast, scalable, pay-per-token APIs for the top frontier models like DeepSeek V3 and Llama 3.3. Fully OpenAI-compatible. Set up in minutes. Scale forever.
What is Inference in Machine Learning? Machine learning projects often encounter roadblocks that can stall or derail progress. One such challenge stems from the data itself. Datasets containing features with very different ranges can create significant problems. This is especially true for algorithms that calculate distances between data points, like k-nearest neighbors, support vector machines, and principal component analysis. To avoid these issues, applying feature scaling in machine learning is crucial before training models on the data. This article will define feature scaling and why it matters.
We’ll explore different methods for scaling features and discuss how to choose the best approach for your specific machine learning project. Inference's AI inference APIs can help you get there faster with reliable, production-ready machine learning models.
What Is Feature Scaling in Machine Learning?
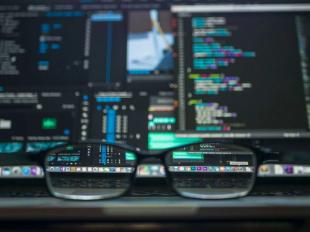
Feature scaling means putting all features of your data within the same range. It is especially relevant when your machine learning models use optimization algorithms or metrics that depend on a distance metric. If feature scaling isn’t done before training machine learning algorithms, the model may be less accurate or take longer to train.
Imagine a dataset with different people's:
- Weights
- Height
Uneven Ranges
For the heights we use meters, which go from 1.51m in the shortest person to 1.97m in the tallest. For the weights, we use kilograms, which go from 48kg in the lightest to 110kg in the heaviest. As you can see, in absolute values, the range of the weights feature is much bigger than the range of the meters feature.
One solution could be to convert the units of the meters to centimeters, but that would just solve this specific scenario, and in many cases, we have more than two features and can’t do this for our whole dataset.
How Feature Scaling Works In Machine Learning
Why is feature scaling important? The following image highlights the importance of feature scaling using the previous height and weight example:
The weight feature dominates this two-variable dataset, as most of our data's variation happens within it. On our X axis, the height variable barely has any variability compared to the weight variable on the Y axis. Any time we use a machine learning algorithm that uses some sort of distance metric, the weight variable will have much more impact than the height variable.
If we scale these features using a Minmaxscaler scaling technique, our weight no longer dominates the feature space, as seen in the following figure:
Our Minmaxscaler has put both features in the 0 to 1 range, allowing them to have the same order of magnitude and eliminating the dominance of the weight variable we had before.
The Benefits of Feature Scaling in Machine Learning
Let’s explore the importance of feature scaling in machine learning in more detail.
Enhancing Model Performance
Feature scaling can significantly enhance the performance of machine learning models. Scaling the features makes it easier for algorithms to find the optimal solution, as the different scales of the features do not influence them.
It can lead to faster convergence and more accurate predictions, especially using algorithms like:
- k-nearest neighbors
- Support vector machines
- Neural networks
Addressing Skewed Data and Outliers
Skewed data and outliers can negatively impact the performance of machine learning models. Scaling the features can help in handling such cases. Transforming the data to a standardized range:
- Reduces the impact of extreme values
- Makes the model more robust
This is particularly beneficial for algorithms that:
- Assume a normal distribution
- Are sensitive to outliers, such as linear regression
Faster Convergence During Training
For gradient descent-based algorithms, feature scaling can speed up the convergence by helping the optimization algorithm reach the minima faster.
Since gradient descent updates the model parameters in steps proportional to the gradient of the error with respect to the parameter, having features on the same scale:
- Allows the algorithm to take more uniform steps towards the optimum
- Reduces the number of iterations needed
Balanced Feature Influence
When features are on different scales, there is a risk that larger-scale features will dominate the model’s decisions, while smaller-scale features will be neglected. Feature scaling ensures that each feature can influence the model without being overshadowed by other features simply because of its scale.
Improved Algorithm Behavior
Certain machine learning algorithms, particularly those that use distance metrics like Euclidean or Manhattan distance, assume all features are centered around zero and have variance in the same order. Without feature scaling, the distance calculations could be skewed, leading to biases in the model and potentially misleading results.
Feature scaling normalizes the range of features so that each one contributes equally to the distance calculations.
Where Feature Scaling in Machine Learning is Applied
As many algorithms like KNN, K-means, etc. use distance metrics to function, any difference in the order of magnitude in the values of our different features will cause the features with the broadest range to dominate the metric, thus taking most of the model's importance.
Because of this, prior to training any algorithm of this kind, we should apply feature scaling techniques to keep our variables within the same range.
Algorithm Sensitivity
The K-Nearest Neighbors (KNN) classification algorithm commonly uses an Euclidean distance metric that performs much better if feature scaling is applied to the data. Feature scaling is also necessary in a K-means clustering algorithm for the same reason.
In a Principal Component Analysis (PCA), feature scaling is essential as we compute the variance under the same unit of measure. Some PCA implementations, like Scikit-learn’s, perform normalization for you, but it is always good to do it beforehand, just in case.
Gradient Descent
If you don’t know what Scikit-Learn is, you can learn all about it with our article: What is Scikit-learn? Any algorithm that learns using gradient descent, like linear or logistic regression, or artificial neural networks, benefits a lot from some sort of feature scaling, both in the speed and the quality of the training.
Algorithm Independence
It is not 100% mandatory to do it like in the previous 3 cases. Algorithms like Decision Trees, Random Forest, or Boosting models don’t care much about the scale of the features, nor do algorithms like Naive Bayes.
It is essential to know that, like any other data pre-processing technique, the scaling algorithms should be fit to just the training data and not the whole data set. Also, always consider your data before using a feature scaling technique.
Feature Scaling Techniques: Pros and Cons
The main feature scaling techniques typically used are standardization and normalization: Normalization scales our features to a predefined range (usually the 0–1 range), independently of the statistical distribution they follow. It uses the minimum and maximum values of each feature in our dataset, making it sensitive to outliers.
Standardization makes our data follow a Normal distribution, usually with a mean of 0 and a standard deviation of 1. It is best to use it when we know our data follows a standard distribution or if we know there are many outliers.
- There is no silver bullet for knowing when to apply each, as it depends on our data and models. As a general rule of thumb, you can follow the following guidelines: Unsupervised learning algorithms usually benefit more from standardization than normalization.
- If your variables follow a normal distribution, then standardization works better.
- Standardization is better if the data presents outliers, as normalization can be hijacked by extremely low or high values in our features. In all other cases, normalization is preferable.
- Despite these rules, we suggest you try both techniques on your data pre-processing pipeline. The results will vary greatly depending on your data and algorithms.
Related Reading
10 Feature Scaling Techniques in Machine Learning
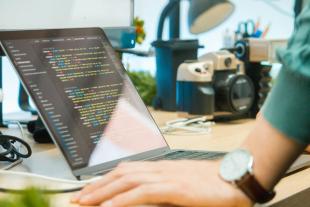
1. Standardization (Z-score normalization)
Standardization transforms data to have zero mean and unit variance. Each feature is scaled independently, making the distribution of each feature centered around zero with a standard deviation of 1. This technique is robust and works well for most machine learning algorithms. It is defined as:
x_standardized = (x - mean(x)) / std(x)
where:
x is the original data point
mean(x) is the mean of the data
std(x) is the standard deviation of the data
Pseudocode:
def standardization(data):
mean = np.mean(data)
std_dev = np.std(data)
standardized_data = (data - mean) / std_dev
return standardized_data
2. Min-Max Scaling
Min-Max scaling, known as normalization, scales features to a specific range (e.g., [0, 1]). It maintains the relative relationship between values and preserves the shape of the distribution. This technique is suitable when features have a known range and there are no significant outliers.
The formula for Min-Max scaling is:
x_scaled = (x - min(x)) / (max(x) - min(x))
where:
x is the original data point
min(x) is the minimum value in the data
max(x) is the maximum value in the data
Pseudocode:
def min_max_scaling(data):
min_val = min(data)
max_val = max(data)
scaled_data = (data - min_val) / (max_val - min_val)
return scaled_data
3. Robust Scaling
Robust scaling is useful when data contains outliers. It scales features using statistics robust to outliers, such as the median and interquartile range (IQR). This technique reduces the impact of extreme values by using percentiles instead of mean and standard deviation. The formula for robust scaling is:
x_scaled = (x - median(x)) / IQR(x)
where:
x is the original data point
median(x) is the median of the data
IQR(x) is the interquartile range of the data (75th percentile - 25th percentile)
Pseudocode:
def robust_scaling(data):
median = np.median(data)
q1 = np.percentile(data, 25)
q3 = np.percentile(data, 75)
iqr = q3 - q1
scaled_data = (data - median) / iqr
return scaled_data
4. Logarithmic Scaling:
Logarithmic scaling transforms data using the logarithm function. It is effective when dealing with highly skewed data or data with exponential growth patterns. Applying the logarithm can help normalize the distribution and reduce the impact of extreme values. The formula for logarithmic scaling is:
x_scaled = log(1+x)
where:
x is the original data point
Pseudocode:
def logarithmic_scaling(data):
scaled_data = np.log1p(data)
return scaled_data
5. Power Transformation:
Power transformation is a versatile technique used to modify the distribution of data. We can address issues such as skewness and unequal variance by applying a power function to each data point. The choice of power function depends on the desired transformation. Common power transformations include:
- Square root
- Logarithmic
- Reciprocal transformations
These transformations can make the data conform more closely to assumptions required by statistical tests or models. The formula for power transformation is:
x_transformed = x^p
where:
x is the original data point
p is the power parameter for the transformation
Pseudocode:
def power_transformation(data, power):
transformed_data = np.power(data, power)
return transformed_data
6. Binning
Binning is dividing continuous variables into smaller, discrete intervals or bins. It is beneficial when dealing with large ranges of data. Binning simplifies data analysis by converting continuous data into categorical data, allowing for more straightforward iInterpretation and analysis
It can be performed by specifying the number of bins or defining the width of each bin. Binning is often used in data preprocessing to create histograms, identify outliers, or develop new features for machine learning algorithms. It does not have a specific mathematical formula associated with it. Pseudocode:
def binning(data, num_bins):
bin_edges = np.linspace(min(data), max(data), num_bins + 1)
binned_data = np.digitize(data, bin_edges)
return binned_data
7. Quantile Transformation
Quantile transformation is a technique that transforms the distribution of a variable to a specified distribution, commonly the standard normal distribution. It involves mapping the original variable values to the corresponding quantiles of the desired distribution.
This transformation helps achieve a more symmetric and uniform distribution, making it suitable for statistical techniques and machine learning algorithms that assume normality. It is particularly valuable when dealing with skewed data. It does not have a specific mathematical formula associated with it. Pseudocode:
def quantile_transform(data):
sorted_data = np.sort(data)
quantiles = np.linspace(0, 1, len(data))
transformed_data = np.interp(data, sorted_data, quantiles)
return transformed_data
8. Unit Vector Scaling
Unit vector scaling, also known as normalization, is a widely used technique to scale the values of a variable to a fixed range, typically between 0 and 1. It involves subtracting the minimum value from each data point and dividing by the range (maximum value minus minimum value).
Normalization ensures that all values are proportionally adjusted to fit within the desired range while preserving the relative relationships between the data points. This technique is particularly helpful when the scale of variables varies widely. The formula for unit vector scaling is:
x_scaled = x / ∥x∥
where:
x is the original data point
∥x∥ represents the Euclidean norm of x Pseudocode:
def unit_vector_scaling(data):
norm = np.linalg.norm(data)
scaled_data = data / norm
return scaled_data
9. Binary Scaling
Binary scaling, or binarization, converts numerical data into binary values. It involves setting a threshold value and assigning 0 to all values below the threshold and 1 to values equal to or above it. Binarization is useful when specific algorithms require binary inputs, such as association rules mining or certain feature selection methods.
It simplifies the data by categorizing it into two classes, which can be advantageous in some scenarios. Binary scaling is more process-oriented and does not have specific mathematical formulas associated with it. Pseudocode:
def binary_scaling(data, threshold):
scaled_data = np.where(data >= threshold, 1, 0)
return scaled_data
10. Max Absolute Scaling
Max Absolute scaling is a data normalization technique that scales the values of a variable to the range of [-1, 1]. It involves dividing each data point by the maximum absolute value among all the data points. Max Absolute scaling preserves the sign of the original values while ensuring they fall within the specified range.
This technique is especially useful when dealing with data that contains outliers that could significantly impact other scaling techniques. The formula for max absolute scaling is: x_scaled = x / max(∣x∣)
where:
x is the original data point
max(∣x∣) represents the maximum absolute value in the Pseudocode:
def max_absolute_scaling(data):
max_val = np.max(np.abs(data))
scaled_data = data / max_val
return scaled_data
Related Reading
Start Building with $10 in Free API Credits Today!
Inference delivers OpenAI-compatible serverless inference APIs for top open-source LLM models, offering developers the highest performance at the lowest cost in the market. Beyond standard inference, Inference provides specialized batch processing for large-scale async AI workloads and document extraction capabilities designed explicitly for RAG applications.
Start building with $10 in free API credits and experience state-of-the-art language models that balance cost-efficiency with high performance.