What Are LLM Embeddings & How to Leverage Them in Real Projects
Published on Apr 21, 2025
Get Started
Fast, scalable, pay-per-token APIs for the top frontier models like DeepSeek V3 and Llama 3.3 . Fully OpenAI-compatible. Set up in minutes. Scale forever.
As machine learning models grow in size and complexity, they require vast data to train effectively. This data is often noisy, unstructured, and incomplete. To make matters worse, model performance can plateau when they encounter new data that differs significantly from their training datasets. LLM embeddings can help with these challenges. By transforming raw data into smaller, more manageable, and structured representations, LLM embeddings help machine learning models acclimate to new information more quickly. Additionally, What is Inference in Machine Learning?
This blog covers how LLM embeddings work, how to generate them, and how AI inference APIs use them to boost model accuracy and fine-tuning efficiency.
What Are LLM Embeddings?
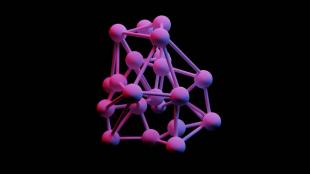
Embeddings are the semantic backbone of LLMs, the gate through which raw text is transformed into vectors of numbers that are understandable by the model. When you prompt an LLM to help debug my code, your words and tokens are transformed into a high-dimensional vector space where semantic relationships become mathematical relationships.
The Versatility of LLM Embeddings
LLM embeddings are vector representations of words, phrases, or entire texts generated by language models. They capture the semantic meaning of the text in a high-dimensional space, allowing for more contextual awareness of the meaning of words. They can be used across NLP tasks without the need for task-specific methods:
- Text classification
- Sentiment analysis
- Information retrieval
- Answering questions
- Machine translation
- Many more
Embeddings vs. One-Hot Encoding
They are also effective in handling large and diverse datasets. Embeddings are unlike one-hot encoding, representing words as sparse vectors with high dimensionality and little meaningful structure. Instead, embeddings map words to dense vectors in a lower-dimensional space.
This mapping is done so semantically similar words are closer together in the embedding space.
How Do LLM Embeddings Work?
Language models are trained on massive datasets, learning patterns and relationships within the text. This training enables the model to understand context, syntax and semantics. Once trained, the model can convert text into numerical vectors. Each vector represents a point in a high-dimensional space where semantically similar texts are closer.
Contextual Understanding in LLM Embeddings
For instance, the words “girl” and “boy” would have vectors that are closer together than “girl” and “banana.” Unlike traditional word embeddings like Word2Vec or GloVe, LLM embeddings take context into account. For example, the word “bank” would have different embeddings in “river bank” and “bank account” scenarios.
Embedding Larger Text Units
LLMs can also generate embeddings for larger text units like sentences and documents. This involves pooling strategies or specialized models designed to capture the meaning of longer texts, using multiple layers of neural networks and attention mechanisms to refine the embeddings.
Key Types of Embeddings
Different types of embeddings help machines process and interpret information more effectively by converting data into meaningful numerical representations. Let’s explore the key types of embeddings and how they power various AI applications:
Word Embeddings
Word embeddings represent individual words as vectors of numbers in a high-dimensional space. These vectors capture semantic meanings and relationships between words, making them fundamental in NLP tasks. By positioning words in such a space, similar words are placed closer together, reflecting their semantic relationships. This allows machine learning models to understand and process text more effectively.
Applications of Word Embeddings
Word embeddings help classify texts, like spam detection or sentiment analysis by understanding the words' context. They enable the generation of concise summaries by capturing the essence of the text. Word embeddings allow models to provide accurate answers based on the context of the query and facilitate the translation of text from one language to another by understanding the semantic meaning of words and phrases.
Sentence and Document Embeddings
Sentence embeddings represent entire sentences as vectors, capturing the context and meaning of the sentence. Unlike word embeddings, which only capture individual word meanings, sentence embeddings consider the relationships between words within a sentence, providing a more comprehensive understanding of the text.
These are used to categorize larger text units, such as sentences or entire documents, making the classification process more accurate. They also help generate summaries by understanding the document's context and key points.
Models can also answer questions based on the context of entire sentences or documents. They improve translation quality by preserving the context and meaning of sentences during translation.
Graph Embeddings
Graph embeddings represent nodes in a graph as vectors, capturing the relationships and structures within the graph. These are particularly useful for tasks that involve network analysis and relational data. For instance, in a social network graph, it can represent users and their connections, enabling tasks like:
- Community detection
- Link prediction
- Recommendation systems
ML models can process and analyze graph data efficiently by transforming the complex relationships in graphs into numerical vectors. One key advantage is their ability to preserve the graph's structural information, which is critical for accurately capturing the relationships between nodes.
Diverse Applications
This capability makes them suitable for a wide range of applications beyond social networks, such as:
- Biological network analysis
- Fraud detection
- Knowledge graph completion
Tools like DeepWalk and Node2Vec have been developed to generate graph embeddings by learning from the graph’s structure, further enhancing the ability to analyze and interpret complex graph data.
Image and Audio Embeddings
Images are represented as vectors by extracting features from them, while audio signals are converted into numerical representations by embeddings. These are crucial for tasks involving visual and auditory data.
Image and Audio Embeddings
Embeddings for images are used in tasks like image classification, object detection, and image retrieval, while those for audio are applied in speech recognition, music genre classification, and audio search. These are potent tools in NLP and machine learning, enabling machines to understand and process various forms of data.
By transforming text, images, and audio into numerical representations, they enhance the performance of numerous tasks, making them indispensable in artificial intelligence.
What Makes a Good Embedding?
When it comes to LLMs, embeddings can be considered the dictionary of their language. Better embeddings allow these models to understand human language and communicate with us. But what makes an embedding technique good? In other words, what makes an embedding ideal?
Key properties and characteristics of LLM embeddings include:
Dimensionality
Embeddings are fixed-size vectors, typically ranging from hundreds to thousands of dimensions. The dimensionality determines how much information each embedding can hold. Higher dimensions can capture more nuances, but also require more computational resources.
Contextuality
The embedding for a word or phrase changes depending on the surrounding text, allowing the model to capture the meaning of words in context rather than in isolation.
Semantic Similarity
Embeddings are designed so that similar words or phrases have identical vectors. For example, the embeddings for “cat” and “dog” will be closer to each other than to “car”. This property helps with tasks like semantic search, clustering and recommendation systems.
Transferability
Embeddings can be used across different tasks without retraining the model from scratch. For instance, embeddings generated by a model trained on a large corpus can be fine-tuned for specific tasks like sentiment analysis or named entity recognition.
Scalability
LLM embeddings can be scaled to accommodate large datasets. They can be computed in batches and stored efficiently, enabling their use in large-scale applications like search engines and recommendation systems.
Sparsity and Density
Embeddings are dense representations, meaning most of the elements in the vector are non-zero. This contrasts with sparse representations like one-hot encoding, where most elements are zero. Dense embeddings capture more information efficiently.
Multi-Modal Capabilities
Advanced LLMs can generate text, images, audio, and other modalities embeddings. These multi-modal embeddings enable the integration of different types of data into a unified representation.
Robustness and Adaptability
LLM embeddings are robust to various linguistic phenomena, such as polysemy (words with multiple meanings) and synonymy (different words with similar meanings). They adapt well to other domains and languages, making them versatile for cross-lingual and cross-domain applications.
Training and Fine-Tuning
Embeddings can be pre-trained on large corpora and then fine-tuned for specific tasks. This pre-training allows the embeddings to capture general linguistic patterns, while fine-tuning enables the embeddings to adapt to the particular requirements of a task.
What is the Difference Between Token and Embedding in LLM?
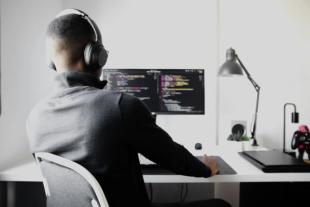
LLM Tokenization
At the time of writing, most people interact with language models through a web-based interface that offers a chat-like experience between the user and the model. You may have noticed that the model doesn’t provide its entire response instantly; instead, it generates the output one token at a time.
Nevertheless, tokens aren’t just how the model creates responses; they also represent how it interprets the input. When you send a text prompt to the model, it is first broken down into tokens.
Tokens
A “token” can represent a word, part of a word, or even a punctuation mark, depending on the tokenizer used. When you give a sentence to a language model, it first breaks down the input into these smaller pieces (tokens).
Tokenization Example: Let’s say you input the sentence:
Input: I love programming.
This sentence will be split into tokens by the tokenizer of the LLM.
Tokens
['I', ' love', ' programming', '.']
Different tokenizers handle tokenization differently. For example, a subword tokenizer could break down certain words into smaller parts:
Example of subword tokens
['I', ' love', ' pro', 'gram', 'ming', '.']
In this case, “programming” is split into subword tokens (‘pro’, ‘gram’, ‘ming’), while “I” and “love” remain whole tokens.
Each token is associated with a unique integer ID, which is how the model understands the text.
Token IDs Example: [72, 104, 3562, 4]
Each token corresponds to a specific number that represents it in the model’s vocabulary.
Embeddings
After the model tokenizes the input text into tokens, it converts each token into a vector of numbers, called an embedding. Embeddings are dense vectors that capture the meaning or context of a token in a continuous vector space.
The model doesn’t work with raw text directly; it works with these embeddings. Each embedding is a multi-dimensional vector (e.g., a 768-dimensional vector) that encodes the semantic meaning of the token.
For example, let’s say the token “love” is represented as the vector:
Mapping Relationships
[0.32, -0.15, 0.78, ...] This vector could have 768 or more dimensions.
Similarly, every token will have its embedding vector. The embeddings allow the model to understand relationships between words, like synonyms or context. For instance, embeddings of related words like “love” and “affection” would be close in this vector space.
How Embeddings Work
When you give a sentence to an LLM, it will:
Tokenize the input text.
- Look up the corresponding embedding vector for each token.
- Feed these embeddings into a series of neural network layers to generate context-aware representations and produce a response.
Example Walkthrough
Let’s take the input sentence:
I love programming.
Tokenization: The sentence is tokenized into
['I', ' love', ' programming', '.']
Embedding Lookup
Each token is mapped to a corresponding embedding vector (simplified here):
I -> [0.01, 0.23, -0.45, ...]
love -> [0.32, -0.15, 0.78, ...]
programming -> [0.56, -0.31, 0.09, ...]
. -> [-0.12, 0.11, 0.03, ...]
Each of these vectors is a numerical representation of the token.
Contextual Processing in Neural Networks
Neural Network Processing
These embeddings are then passed through the model layers, where they are processed about each other. The model uses this information to understand the sentence contextually. For example, it understands that “love” refers to a positive sentiment and that “programming” is an activity.
Output Generation
The model then generates tokens in response, using embeddings to ensure the output is semantically and contextually appropriate.
Example of Input and Output
Input Text:
“I love programming.”
Tokenized Input:
['I', ' love', ' programming', '.']
2. Token IDs:
[72, 104, 3562, 4]
Embedding Vectors
These are multi-dimensional vectors (simplified here for illustration):
[
[0.01, 0.23, -0.45, ...], # for 'I'
[0.32, -0.15, 0.78, ...], # for ' love'
[0.56, -0.31, 0.09, ...], # for ' programming'
[-0.12, 0.11, 0.03, ...] # for '.'
]
Generated Output
If the model is asked to complete the sentence or generate a response, it might produce:
Output Text: “It’s a great skill to have.”
Generated Tokens
[' It', "'s", ' a', ' great', ' skill', ' to', ' have', '.']
Generated Token IDs:
[27, 8, 10, 231, 645, 18, 76, 4]
Summary
Tokens are the basic units of input and output for LLMs, representing words or subwords.
Embeddings are vectors that translate tokens into a form the model can understand, encoding semantic meaning in a continuous space. The model uses these embeddings to process text and generate meaningful responses, always working with tokens and their embeddings rather than raw text.
Related Reading
10 Main Approaches to LLM Embeddings
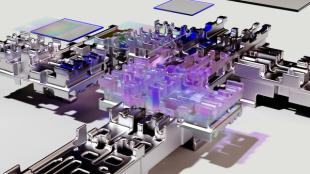
Word embeddings serve as the foundational layer of LLM embeddings. These vector representations capture how words are used in real data, translating human language into a numerical format that machine learning algorithms can understand. Word embeddings reduce the dimensionality of textual data, allowing models to learn more efficiently.
1. Word2Vec: Predicting Words and Their Contexts
Word2Vec predicts a word given its context (CBOW) or the context given a word (Skip-gram). For example, in the phrase “The bird sat in the tree,” Word2Vec can learn that “bird” and “tree” often appear in similar contexts, capturing their relationship. This is useful for tasks like word similarity and analogy detection.
2. GloVe: Understanding Word Relationships
GloVe (Global Vectors for Word Representation) uses matrix factorization techniques on the word co-occurrence matrix to find word embeddings. For instance, GloVe can learn that “cheese” and “mayo” are related to “sandwich” by analyzing the co-occurrence patterns across a large corpus.
This approach is excellent for applications like semantic search and clustering that need to understand broader relationships among words.
3. FastText: Considering Subword Information
FastText, an extension of Word2Vec by Facebook, considers subword information, making it effective for morphologically rich languages. It represents words as bags of character n-grams, which helps understand rare words and misspellings. For example, it can recognize that “running” and “runner” share a standard subword structure.
4. Contextualized Word Embeddings: Going Beyond Static Representations
Static word embeddings generate fixed representations for words, regardless of context. Nevertheless, contextualized embeddings dynamically produce word vectors that consider the surrounding text. This allows for more nuanced and accurate representations that capture subtle semantic differences.
5. ELMo: A First Step in Contextualized Embeddings
Embeddings from Language Models (ELMo) generate word representations that are functions of the entire input sentence, capturing context-sensitive meanings. For example, the word “bark” will have different embeddings, such as “The dog began to bark loudly” versus “The tree’s bark was rough,” depending on the surrounding words.
6. BERT: A Breakthrough in Contextualized Embeddings
BERT (Bidirectional Encoder Representations from Transformers) pre-trains deep bidirectional representations by jointly conditioning on both left and right context in all layers. For example, in the sentence “She went to the bank to deposit money,” BERT uses the preceding words “She went to the” and the following words “to deposit money” to determine that “bank” refers to a financial institution, not a riverbank.
7. GPT: A Unidirectional Approach to Contextualized Embeddings
GPT (Generative Pre-trained Transformer) by OpenAI uses a unidirectional approach, which generates embeddings that consider the left context. For example, in a sentence like “The weather today is,” GPT uses the preceding words to predict that “sunny” or “rainy” might follow. This works well for tasks like text generation and completion, where sequence is essential.
8. Sentence and Document Embeddings: For Larger Text Structures
Embeddings aren’t limited to words. We can generate embeddings for sentences, paragraphs, and entire documents to capture their meanings and facilitate efficient comparisons.
9. Universal Sentence Encoder: A Transformer for Sentence Embeddings
Using a transformer or deep averaging network, the Universal Sentence Encoder (USE) encodes sentences into high-dimensional vectors. For example, the sentences “The quick brown fox jumps over the lazy dog” and “A swift auburn fox leaps over a sleepy canine” would have similar embeddings because they convey the same meaning.
10. Sentence-BERT: Fine-Tuning BERT for Sentence Embeddings
Sentence-BERT (SBERT) fine-tunes BERT on sentence-pair regression tasks to produce meaningful sentence embeddings. For instance, determining that “How do I reset my password?” is similar in meaning to “What is the process to change my password?”. This capability is excellent for applications like FAQ matching and paraphrase detection.
11. Doc2Vec: Extending Word2Vec for Document Embeddings
Doc2Vec extends Word2Vec to generate embeddings for larger chunks of text, like paragraphs or documents. For example, it can represent an entire news article about a recent election as a single vector, enabling efficient comparison and grouping of similar articles.
12. InferSent: A Supervised Approach to Sentence Embeddings
InferSent, developed by Facebook, is a sentence embedding method that uses supervised learning. It employs a bidirectional LSTM with max-pooling trained on natural language inference (NLI) data to produce general-purpose sentence representations. For instance, InferSent can create embeddings for customer reviews, allowing a company to analyze and compare feedback across different products.
13. Universal Sentence Encoder: A Transformer for Sentence Embeddings
Using a transformer or deep averaging network, the Universal Sentence Encoder (USE) encodes sentences into high-dimensional vectors. For example, the sentences “The quick brown fox jumps over the lazy dog” and “A swift auburn fox leaps over a sleepy canine” would have similar embeddings because they convey the same meaning.
14. Transformer-based Embeddings: The Next Generation of LLM Embeddings
GPT-3 (Generative Pre-trained Transformer 3) uses a large-scale transformer model to generate embeddings by predicting the next word in a sequence. This approach can create high-quality embeddings that improve performance on various natural language processing tasks.
15. Specialized Embeddings: Tailoring Representations to Specific Domains
ClinicalBERT, SciBERT, and other specialized embeddings fine-tune BERT on domain-specific corpora to create embeddings tailored for specific fields like healthcare or scientific literature. These approaches help models better understand target areas' unique vocabulary, structures, and nuances to improve performance on specialized data tasks.
16. Combined Approaches: Hybrid Models for LLM Embeddings
Embedding methods and models can also be combined for improved performance. Hybrid models, for example, mix different types of embeddings or models (e.g., combining word embeddings with contextualized embeddings) to leverage their complementary strengths.
Related Reading
- What is Quantization in Machine Learning
- Batch Learning vs. Online Learning
- Feature Scaling in Machine Learning
In music classification, embeddings can capture the features of different musical notes and sequences, enabling the model to classify the music into other genres. In audio generation, embeddings can capture the features of different sounds, allowing the model to generate new sounds or create ones consistent with the existing ones.
In the video domain, embeddings are used in object detection, action recognition, and video generation tasks. In object detection, embeddings can capture the features of different objects in the video, allowing the model to identify and locate these objects.
Using Embeddings for Action Recognition and Video Generation
In action recognition, embeddings can capture the features of different actions, enabling the model to recognize and classify these actions. In video generation, embeddings can capture the features of various frames, allowing the model to generate new frames consistent with the previous ones, resulting in a coherent video.
Transforming Raw Data for Model Understanding and Generation
In all these applications, embeddings serve as the bridge between the raw data and the model, transforming the data into a form the model can understand and learn from. This enables the model to recognize patterns in the data and generate new data that follows these patterns, thereby achieving the desired task.
E-commerce Personalized Recommendations
Platforms use these vector representations to offer personalized product suggestions. By representing products and users as vectors in a high-dimensional space, e-commerce platforms can analyze user behavior, preferences, and purchase history to recommend products that align with individual tastes.
This method enhances the shopping experience by providing relevant suggestions, driving sales, and customer satisfaction. For instance, embeddings help platforms like Amazon and Zalando understand user preferences and deliver tailored product recommendations.
Chatbots and Virtual Assistants
Application and Implementation of LLM Embedding
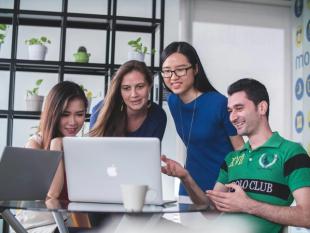
Vector embeddings have become an integral part of numerous real-world applications, enhancing the accuracy and efficiency of various tasks. Here are some compelling examples showcasing their power:
Audio and Video Processing
In the audio domain, embeddings are used in tasks like:
- Speech recognition
- Music classification
- Audio generation
In speech recognition, the audio input is converted into a spectrogram, which is then transformed into embeddings. These embeddings capture the unique characteristics of the speaker’s voice and the words they’re saying, allowing the model to transcribe the audio accurately.
Applying Embeddings in Music and Audio Tasks
Embeddings enable better understanding and processing of user queries. Modern chatbots and virtual assistants, such as those powered by GPT-3 or other large language models, utilize these to comprehend the context and semantics of user inputs. This allows them to generate accurate and contextually relevant responses, improving user interaction and satisfaction. For example, chatbots in customer support can efficiently resolve queries by understanding the user’s intent and providing precise answers.
Social Media Sentiment Analysis
Companies analyze social media posts to gauge public sentiment. By converting text data into vector representations, businesses can analyse sentiment to understand public opinion about their products, services, or brand. This analysis helps track customer satisfaction, identify trends, and make informed marketing decisions. Tools powered by embeddings can scan vast amounts of social media data to detect positive, negative, or neutral sentiments, providing valuable insights for brands.
Healthcare Applications
Embeddings assist in patient data analysis and diagnosis predictions. In the healthcare sector, they analyze patient records, medical images, and other health data to diagnose diseases and predict patient outcomes.For instance, specialized tools like Google’s Derm Foundation focus on dermatology, enabling accurate analysis of skin conditions by identifying critical features in medical images. These help doctors make informed decisions, improving patient care and treatment outcomes.
The Transformative Power of Embeddings Across Industries
These examples illustrate the transformative impact of embeddings across various industries, showcasing their ability to enhance personalization, understanding, and analysis in diverse applications. By leveraging this tool, businesses can unlock deeper insights and deliver more effective solutions to their customers.
Considerations for Choosing an Embedding Approach
- Task Requirements: Choose based on the specific needs of your NLP task (e.g., word-level vs. sentence-level understanding).
- Computational Resources: Some models (like BERT or GPT-3) require significant computational power.
- Data Availability: Consider data availability for pre-training or fine-tuning your embeddings.
- Interpretability: Simpler models like Word2Vec might be easier to interpret than complex transformer-based models. Multiple solutions can help you get started, from open-source LLM embeddings tools to LLM embedding databases.
LLM Embeddings and AI Pipelines
LLM Embeddings are part of the AI pipeline in three main ways:
- Integrations: Embeddings can be integrated throughout AI pipelines as inputs to various stages. For instance, they might feed into further neural network layers, be part of a feature extraction process for clustering algorithms, or be used directly in similarity comparisons for recommendation systems.
- Cost Optimization: LLM embeddings use lower-dimensional data. This often means faster training times and less computational overhead than handling sparse, high-dimensional data like one-hot encoded vectors.
- Robust Deployment: Models built on LLM embeddings are generally more robust. This helps deploy the model into real-world environments more successfully.
Related Reading
Start Building with $10 in Free API Credits Today!
Inference delivers OpenAI-compatible serverless inference APIs for top open-source LLM models, offering developers the highest performance at the lowest cost in the market. Beyond standard inference, Inference provides specialized batch processing for large-scale async AI workloads and document extraction capabilities designed explicitly for RAG applications.
Start building with $10 in free API credits and experience state-of-the-art language models that balance cost-efficiency with high performance.