What is Domain Adaptation and Why It Matters for Real-World AI
Published on Apr 22, 2025
Get Started
Fast, scalable, pay-per-token APIs for the top frontier models like DeepSeek V3 and Llama 3.3 . Fully OpenAI-compatible. Set up in minutes. Scale forever.
Machine learning models are rapidly gaining traction in industries where they can boost efficiency and provide valuable insights. Nevertheless, many of these applications require the algorithms to work accurately in real-world environments where data can vastly differ from the information used to train the model. For example, a model that detects images of tumors may need to be able to identify abnormal tissues in scans from different hospitals using different imaging equipment and protocols. While the solution may seem simple, retrain the model on new data, this process can be inefficient, costly, and time-consuming. Fortunately, domain adaptation can help researchers build machine learning models that perform reliably across diverse real-world environments, without needing to retrain from scratch for every new dataset. This article will offer valuable insights into the process of domain adaptation and how it can address your goals. Additionally, What is Inference in Machine Learning?
One tool to help you achieve your objectives is Inference's AI inference APIs. With powerful Domain Adaptation solutions, you can fine-tune models to adapt quickly to new datasets, reduce retraining time, and accelerate real-world deployment.
What Is Domain Adaptation and Why Is It Important?
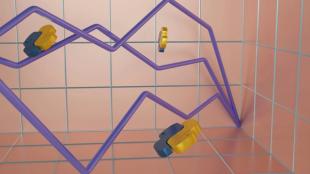
Deep Learning algorithms are the go-to choice for engineers to solve different kinds of Computer Vision problems, from classification and segmentation to object detection and image retrieval. But there are two main problems associated with them.
Neural networks require a lot of labeled data for training, which is laborious to annotate manually. Secondly, a trained deep learning model performs well on test data only if it comes from the same data distribution as the training data.
A dataset created by photos taken on a mobile phone has a significantly different distribution from a high-end DSLR camera. Traditional transfer learning methods fail.
The Bottleneck of Retraining
Thus, for every new dataset, we need to annotate the samples and then re-train the deep learning model to adapt to the new data. Training a sizable Deep Learning model with datasets as big as the ImageNet dataset even once takes a lot of computational power (model training may go on for weeks), and training them again is infeasible.
Bypassing Retraining for Efficient Model Transfer and Adaptation
Domain Adaptation is a method that tries to address this problem. Using domain adaptation, a model trained on one dataset does not need to be re-trained on a new dataset. Instead, the pre-trained model can be adjusted to give optimal performance on this new data.
This saves a lot of computational resources, and in techniques like unsupervised domain adaptations, the new data does not need to be labeled.
What is Domain Adaptation?
Domain adaptation is a subfield of machine learning and computer vision that enables models to generalize across different domains. In computer vision, a domain can be understood as a specific distribution of images or visual data, characterized by unique features such as:
- Lighting conditions
- Backgrounds
- Object appearances
- Camera angles
The core objective of domain adaptation is to adapt a model trained on a source domain (where abundant labeled data is available) to perform well on a target domain (where labeled data is scarce or absent), despite differences in data distribution between the two.
Successful Domain AdaptationDomain adaptation is significant because it can mitigate dataset bias and improve the generalizability of computer vision models. Dataset bias occurs when a model trained on a specific dataset (source domain) performs poorly on data from different distributions (target domains) due to reliance on features that are not universally applicable.
This challenge is particularly acute in real-world applications where environmental conditions, such as lighting and weather, can vary dramatically. By leveraging domain adaptation techniques, developers can create models that maintain high accuracy and reliability across diverse settings, reducing the need for costly data labeling efforts in each new environment.
Theoretical Foundations of Domain Adaptation
Definition of Source and Target Domains
In domain adaptation, the source domain refers to the original dataset or environment where a model has been trained, characterized by a specific distribution of features and labels. Conversely, the target domain denotes the new dataset or environment to which the model needs to be adapted, often lacking extensive labeled data.
The source and target domains differ in their data distributions, a disparity that domain adaptation techniques aim to bridge.
The Shift in Data Distribution and Its Impact on Model Performance
A fundamental challenge in domain adaptation is the shift in data distribution between the source and target domains, also known as domain shift. This shift can occur due to differences in lighting conditions, backgrounds, sensor characteristics, or object appearances, leading to a degradation in model performance when applied to the target domain.
Domain adaptation seeks to understand and minimize the impact of this shift, enabling the transfer of learned features and models from the source domain to perform accurately in the target domain.
Domain Discrepancy Measures
Several domain discrepancy measures have been developed to quantify the difference between source and target domains. Maximum Mean Discrepancy (MMD) and Wasserstein distance are prominent examples. MMD measures the distance between the mean embeddings of features in the source and target domains, aiming to minimize this distance for effective domain adaptation.
Wasserstein distance, derived from optimal transport theory, measures the cost of transporting mass to transform the distribution of the source domain into that of the target domain. These metrics help in designing algorithms that effectively reduce domain discrepancies.
Transferability and Domain-Invariant Features
Transferability refers to the ability of features learned from the source domain to be useful in the target domain. A central goal in domain adaptation is to identify and enhance the transferability of domain-invariant features, relevant and standard attributes across both domains.
Generalizing Across Domains
By focusing on these features, domain adaptation techniques strive to build models that generalize well to new environments, regardless of the domain shift. Identifying such features often involves techniques that encourage the model to ignore domain-specific variations, concentrating instead on the underlying patterns useful for performing the task in both domains.
What is the Difference Between Domain Adaptation and Transfer Learning?
Domain adaptation and transfer learning are related concepts in machine learning, but they differ. Domain adaptation focuses on improving a model's performance when applied to different but related data domains by leveraging knowledge from a source domain.
Transfer learning, on the other hand, is a broader concept that involves transferring knowledge learned from one task or domain to another, potentially unrelated task or domain, to improve a model's performance.
Related Reading
7 Types of Domain Adaptation
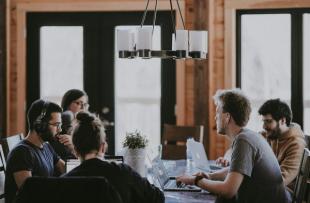
Domain adaptation techniques vary depending on the similarity between source and target domains and the availability of labeled data. Depending on factors like the available annotations of the target domain data, the nature of the source and target domain feature spaces, and the path traversing which domain adaptation is achieved, domain adaptation methods can be categorized into several types.
1. Supervised Domain Adaptation
Strategies Using Labeled Data in Target Domain
Supervised domain adaptation techniques rely on the availability of some labeled data in the target domain, though significantly less than in the source domain.
Fine-Tuning with Target Labels
These strategies often involve fine-tuning a pre-trained model on the target domain’s labeled data to adjust the model weights, ensuring the model performs well on both the source and target data. Techniques such as feature alignment and instance reweighting are employed to minimize the domain gap while effectively leveraging the scarce labeled data in the target domain.
Limitations and Applicability
While supervised domain adaptation can achieve high accuracy when some target domain labels are available, its applicability is limited by the need for labeled data in the target domain. Labeling data can be expensive and time-consuming, particularly in specialized fields.
The success of supervised domain adaptation depends on the availability and quality of labeled data in the target domain.
2. Unsupervised Domain Adaptation
Approaches for Scenarios Lacking Labeled Target Data
Unsupervised domain adaptation techniques are designed for scenarios where no labeled data is available in the target domain. These methods leverage the abundant labeled data in the source domain and the unlabeled data in the target domain to reduce the domain discrepancy.
Adversarial and Self-Ensembling Strategies
Techniques such as adversarial training, where a model is trained to perform the primary task and be unable to distinguish between source and target domain data, and self-ensembling, where predictions on target domain data are made consistent over different epochs, are commonly used.
Forcing Domain Invariance
Prominent Methods (e.g., Adversarial Training, Self-ensembling): Adversarial training involves a domain discriminator that is trained to distinguish between source and target domain features, while the feature extractor is trained to fool the discriminator, leading to domain-invariant feature representations.
Self-ensembling, on the other hand, uses consistency training, where the model’s predictions on unlabeled target domain data are made consistent under different perturbations or model parameters.
3. Semi-supervised Domain Adaptation
Leveraging Limited Labeled Data in Target Domain Alongside Source Domain Data
Semi-supervised domain adaptation methods exploit a small amount of labeled data in the target domain in conjunction with a large amount of labeled data in the source domain.
This approach typically involves strategies that balance the adaptation using both the labeled target domain data for direct supervision and the unlabeled target domain data for aligning the data distributions.
Techniques and Success Stories
Techniques include combining the strengths of supervised and unsupervised domain adaptation methods, such as using labeled target data for fine-tuning and unlabeled target data for domain alignment through adversarial methods or entropy minimization.
Success stories often highlight significant improvements in model performance on the target domain, demonstrating the efficacy of leveraging even a small amount of labeled data in the target domain.
4. Self-supervised Learning for Domain Adaptation
Utilizing Inherent Data Structure for Domain Adaptation
Self-supervised learning approaches for domain adaptation focus on exploiting the data's inherent structure to learn useful representations without relying on external labels.
By designing pretext tasks, such as predicting a part of the data from another part or reconstructing the input data, models can learn beneficial features for the adaptation task.
Recent Advances and Methodologies
Recent advances include sophisticated pretext tasks that are more aligned with the downstream tasks and innovative network architectures that facilitate better representation learning for domain adaptation. These methodologies emphasize learning domain-invariant and task-relevant features in an unsupervised manner, paving the way for more effective adaptation strategies.
5. Deep Learning Models for Domain Adaptation
Convolutional Neural Network (CNN) Based Approaches Modifications to CNN Architectures for Domain Adaptation: Convolutional Neural Networks (CNNs) form the backbone of many computer vision tasks because they capture hierarchical feature representations from images.
Tailoring Convolutional Networks
For domain adaptation, CNN architectures are often modified to minimize the domain discrepancy at various feature levels. Techniques such as adding domain adaptation layers, employing gradient reversal layers to confuse domain classifiers, and integrating attention mechanisms to focus on domain-invariant features are examples of how CNNs are tailored for domain adaptation.
Case Studies and Results
Numerous studies have demonstrated the effectiveness of modified CNN architectures in domain adaptation tasks. For instance, adapting CNNs for cross-domain image classification has shown significant improvements in accuracy when transferring knowledge from one dataset to another, such as from synthetic to real-world images.
These case studies highlight the practical benefits and challenges of applying CNN-based approaches to domain adaptation, offering insights into strategies for successful adaptation.
6. Generative Adversarial Networks (GANs) in Domain Adaptation
Using GANs for Generating Domain-invariant Features
Generative Adversarial Networks (GANs) have emerged as a powerful tool for domain adaptation by generating indistinguishable features or images across domains.
Forging Domain-Invariant Features with GANs
In this context, the adversarial process involves training a generator to produce domain-invariant features while training a discriminator to distinguish between the source and target domain features. Over time, the generator learns to create features that the discriminator cannot differentiate, leading to effective domain adaptation.
Translating Styles for Effective Cross-Domain Learning
Examples of Successful Implementations: Applications of GANs in domain adaptation include image-to-image translation tasks where images from the source domain are translated into the style of the target domain without changing their underlying content, thereby facilitating model training on the target domain data.
Such implementations have proven successful in applications ranging from enhancing the robustness of object recognition systems to enabling cross-domain semantic segmentation.
7. Transformer Models and Domain Adaptation
Exploring the Role of Transformers in Cross-domain Tasks
More recently, transformer models, known for their success in natural language processing, have been adapted for computer vision tasks. Their ability to model long-range dependencies and capture global context makes them suitable for domain adaptation, where capturing domain-invariant contextual information is crucial.
Researchers are exploring how transformers' self-attention mechanism can be leveraged to focus on relevant features across domains, enhancing domain adaptation efforts.
Potential Advantages and Research Directions
The potential advantages of using transformer models in domain adaptation include their flexibility in handling varying input sizes and their efficacy in modeling complex data relationships.
Ongoing research investigates how transformers can be integrated with existing domain adaptation techniques, such as adversarial training and self-supervision, to further improve their ability to generalize across domains.
These investigations open new avenues for leveraging transformer models' strengths to overcome the challenges posed by domain shifts in computer vision tasks.
Related Reading
- What is Quantization in Machine Learning
- Batch Learning vs. Online Learning
- Feature Scaling in Machine Learning
How Domain Adaptation Works & Its Applications
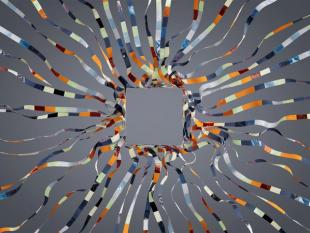
Feature Alignment: The Cornerstone of Domain Adaptation
Feature alignment techniques like Maximum Mean Discrepancy (MMD) and Correlation Alignment (CORAL) work to minimize distributional discrepancies between source and target domains. This means that domain adaptation can help reduce the difference between two domains, enabling a model to better generalize to the target domain.
By aligning features, models learn to focus on similarities between domains, effectively improving performance on the target domain without direct access to its labeled data.
Adversarial Training: Forging Domain-Invariant Features
Rather than working to align source and target domain feature distributions, adversarial training approaches take inspiration from Generative Adversarial Networks (GANs) to help models learn domain-invariant representations. For example, the Adversarial Discriminative Domain Adaptation (ADDA) method employs a discriminator to distinguish between source and target domain features, pushing the model to produce indistinguishable, domain-agnostic features. This not only enhances model generalization across domains but also introduces robustness against overfitting to the source domain's specific characteristics.
Transferable Features: Bridging Domains
Domain adaptation techniques also help identify and leverage features that hold relevance across both source and target domains. Utilizing domain adaptation techniques to discern these transferable features ensures that the model retains its efficacy even when applied to data with different distributions.
The ability to identify transferable features underpins the success of domain adaptation, enabling models to leverage pre-existing knowledge and apply it to new, related tasks.
Iterative Model Adjustment in Classification Tasks
Domain adaptation involves iteratively adjusting the model to minimize prediction error in the target domain. Techniques like pseudo-labeling use the model's predictions on the target domain to iteratively refine its understanding, bridging the gap between the source and target data distributions.
Through continuous refinement, the model achieves improved accuracy on the target domain, effectively adapting to the new data environment.
Domain-Invariant Feature Learning
Domain-invariant feature learning aims to extract features that are robust to changes across domains. Deep learning architectures, particularly those involving feature extraction layers, are optimized to disregard domain-specific noise and focus instead on the underlying patterns common to both domains.
This process improves model generalization across domains, enhancing their applicability and performance in varied real-world scenarios.
Leveraging Deep Learning Architectures
Convolutional Neural Networks (CNNs) and Generative Adversarial Networks (GANs) stand out for their ability to facilitate effective domain adaptation. These architectures play a crucial role in feature extraction and adversarial training, respectively, contributing to developing domain-invariant models.
Utilizing such architectures ensures that domain adaptation methods can be seamlessly integrated into existing deep learning models, maximizing their adaptability and effectiveness.
The Importance of Evaluation Metrics
Specific metrics are vital for assessing the performance of adapted models on the target domain. Metrics such as domain adaptation accuracy, transfer loss, and domain discrepancy measures provide insights into the effectiveness of the adaptation process.
These metrics guide the model optimization process and offer a quantifiable measure of how well the model has adapted to the target domain, ensuring the practical utility of domain adaptation techniques.
Start Building with $10 in Free API Credits Today!
Inference delivers OpenAI-compatible serverless inference APIs for top open-source LLM models, offering developers the highest performance at the lowest cost in the market. Beyond standard inference, Inference provides specialized batch processing for large-scale async AI workloads and document extraction capabilities designed explicitly for RAG applications.
Start building with $10 in free API credits and experience state-of-the-art language models that balance cost-efficiency with high performance.