How to Design an AI Infrastructure Ecosystem for Speed, Scale, & Reliability
Published on Apr 18, 2025
Get Started
Fast, scalable, pay-per-token APIs for the top frontier models like DeepSeek V3 and Llama 3.3. Fully OpenAI-compatible. Set up in minutes. Scale forever.
As AI adoption rises, many organizations face challenges deploying AI models into production. For instance, a recent report revealed that 83% of AI projects have stalled and failed to deliver business value. AI Inference vs Training plays a crucial role in overcoming these hurdles, as understanding the differences helps optimize resource allocation and performance. Creating an AI infrastructure ecosystem to support AI model deployment, operation, and management can help teams overcome these challenges. This article will offer valuable insights to help you build an AI infrastructure ecosystem that seamlessly scales, accelerates model deployment, and ensures reliable performance, empowering your teams to build and run AI applications efficiently.
One solution that can help you achieve your objectives is AI inference APIs. These tools can simplify and streamline how you deploy AI models, enhancing the performance and efficiency of your AI infrastructure ecosystem.
What is an AI Infrastructure?
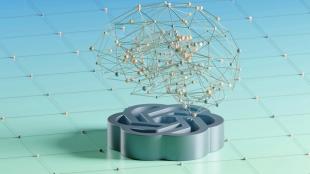
AI infrastructure encompasses the hardware, software, and networking elements that empower organizations to develop, deploy, and manage artificial intelligence (AI) projects effectively. It is the backbone of any AI platform, providing the foundation for machine learning algorithms to process vast amounts of data and generate insights or predictions.
A strong AI infrastructure is crucial for organizations to implement artificial intelligence. The infrastructure supplies the essential resources for developing and deploying AI initiatives, allowing organizations to harness the power of machine learning and big data to obtain insights and make data-driven decisions.
Why AI Infrastructure Ecosystems Matter
The importance of AI infrastructure lies in its role as a facilitator of successful AI and machine learning (ML) operations, acting as a catalyst for innovation, efficiency, and competitiveness.
Here are some key reasons why AI infrastructure is so essential:
Performance and Speed
A well-designed AI infrastructure leverages high-performance computing (HPC) capabilities, such as:
- GPUs
- TPUs
To perform complex calculations in parallel. This allows machine learning algorithms to process enormous datasets swiftly, leading to faster model training and inference.
Speed is critical in AI applications like real-time analytics, autonomous vehicles, or high-frequency trading, where delays can lead to significant consequences.
Scalability
As AI initiatives grow, the volume of data and the complexity of ML models can increase exponentially. A robust AI infrastructure can scale to accommodate this growth, ensuring organizations can handle future demands without compromising performance or reliability.
Collaboration and Reproducibility
AI infrastructure fosters collaboration by providing a standardized environment where data scientists and ML engineers can:
- Share
- Reproduce
- Build upon each other's work
MLOps practices and tools that manage the end-to-end lifecycle of AI projects facilitate this, increasing overall productivity and reducing time to market.
Security and Compliance
With increasing concerns over data privacy and regulatory requirements, a robust AI infrastructure ensures the secure handling and processing of data. It can also help enforce compliance with applicable laws and industry standards, mitigating potential legal and reputational risks.
Cost-Effectiveness
Although building an AI infrastructure might require a substantial initial investment, it can result in significant cost savings over time. By optimizing resource utilization, reducing operational inefficiencies, and accelerating time to market, an effective AI infrastructure contributes to a better return on investment (ROI) in AI projects.
Related Reading
- MLOps Architecture
- MLOps Best Practices
- AI Learning Models
- Machine Learning Best Practices
- Model Inference
- AI Infrastructure
6 Key Components of AI Infrastructure
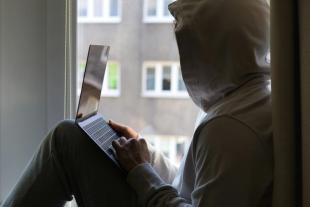
An efficient AI infrastructure gives ML engineers and data scientists the resources to create, deploy, and maintain their models. Here are the primary components of a typical AI technology stack:
1. Computational Power: The Hardware Behind AI Systems
Computational power provides the resources that AI systems use to function, akin to how power keeps a city running. In the case of AI, this power comes from hardware like:
- GPUs
- TPUs
- Other accelerators
Thanks to their parallel processing capabilities, these chips are critical for executing AI workloads effectively.
The Role of TPUs and Cloud Computing in Scaling AI Workloads
TPUs, purpose-built by Google as custom ASICs, are like powerhouses, accelerating machine learning workloads by efficiently handling computational requirements. But the power doesn’t stop at the hardware level.
Advanced techniques like multislice training facilitate large-scale AI model training, which can scale across tens of thousands of TPU chips. And then we have cloud computing.
As organizations need to scale their computational resources up or down as needed, they increasingly rely on cloud-based hardware to offer flexibility and cost-effectiveness for AI workloads. It’s like having a power grid that can deliver just the right amount of electricity when needed.
2. Networking and Connectivity Frameworks: The Highways of AI
A city cannot function without efficient connectivity, nor can AI systems. Networking is central to AI infrastructure, supporting data transfer between storage systems and locations where processing occurs.
High-bandwidth, low-latency networks are crucial, providing rapid data transfer and processing that is key to AI system performance. It’s like the city’s transportation network, ensuring that data, the city's lifeblood, flows smoothly and efficiently.
3. Data Handling and Storage Solutions: Where AI Keeps Its Knowledge
AI systems require robust data storage and management solutions to handle labeled data. These solutions efficiently handle the high volumes of data necessary for training and validating models. Storage options for AI data encompass:
- Databases
- Data warehouses
- Data lakes
Which can be stationed on-premises or hosted on cloud services, offering versatility and scalability. But this isn’t a haphazard process.
Just as a city planner needs to strategically plan the location and design of storage facilities, implementing a data-driven architecture from the initial design phase is critical for the success of AI systems.
4. Data Processing Frameworks: Making Sense of Raw Data
Data processing frameworks play a vital role in acting like the city’s factories, taking in raw data and producing valuable insights. These frameworks are pivotal for handling large datasets and performing complex transformations, enabling distributed processing to perform tasks that expedite data preparation.
But it’s not just about processing data. These frameworks also support distributed computing, allowing the parallelization of AI algorithms across multiple nodes, enhancing resource utilization, and expediting model training and inference. In-memory databases and caching mechanisms reduce latency and improve data access speeds.
5. Security and Compliance: Keeping AI Systems Safe and Sound
Just as a city needs a police force and a set of laws to ensure safety and order, artificial intelligence programs need robust security measures and adherence to regulatory standards. AI platforms can be susceptible to various security threats, such as:
- Data poisoning
- Model theft
- Inference attacks
- The development of polymorphic malware
But it’s not just about security. Compliance plays a crucial role, too. AI systems significantly impact privacy and data protection, posing challenges like informed consent and surveillance concerns. International coverage of AI legal issues features in policies from the:
- United Nations
- OECD
- Council of Europe
- The European Parliament
Acknowledging the significance of human rights and human language in AI development and deployment. AI infrastructure must ensure secure data handling and compliance with laws and industry standards to diminish legal and reputational risks.
6. Machine Learning Operations: The Backbone of AI Efficiency
AI systems require Machine Learning Operations (MLOps) for efficient problem-solving. MLOps involves workflow practices that ensure version control for models, automated training, and deployment pipelines, including unsupervised learning techniques, model performance tracking, and collaboration between different roles.
Automation plays a critical role in MLOps, enabling version control, orchestrating automated pipelines, and efficiently managing machine learning environments' scaling, setup, and maintenance. Continuous evaluation metrics track models' performance, ensuring models' effectiveness over time. Integrating MLOps with DevOps security practices and tools, combined with the adoption of CI/CD, enables the automation of build, test, and deployment processes, making the development of AI models more cohesive and efficient.
Related Reading
- AI Infrastructure
- MLOps Tools
- AI as a Service
- Machine Learning Inference
- Artificial Intelligence Cost Estimation
- AutoML Companies
- Edge Inference
- LLM Inference Optimization
Insights from the First Annual AI Infrastructure Ecosystem Report
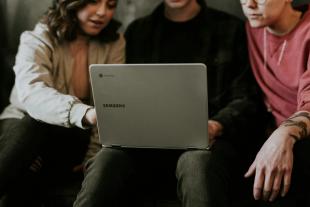
Agents, LLMs, and the New Wave of Smart Apps
When we think of agents, iconic figures like James Bond or Jason Bourne might come to mind:
- Capable
- Autonomous
- Stylish
But after ChatGPT’s release, a new class of agents has emerged: AI agents.
These are intelligent systems capable of interacting with their environments autonomously or semi-autonomously. While the definition is still evolving, at its core, an agent is autonomous software designed to pursue specific goals, whether in:
- Digital spaces
- Physical world
- Or both
Equipped with “sensors” to perceive and “actuators” to respond, these agents might operate through APIs like a language model does, or physically through robotic tools like grippers or LIDAR-enabled navigation.
The Power and Pitfalls of LLM-Driven Agents
Large language models (LLMs) like ChatGPT and GPT-4, built on the influential Transformer architecture, have redefined what’s possible for AI agents. For the first time, these models have the capabilities that were out of reach for earlier systems and serve as:
- Flexible
- General-purpose “brains”
- Capable of tasks ranging from:
- Planning and reasoning
- Decision-making and question answering
LLMs have well-documented limitations. They can “hallucinate”, confidently generate false or misleading information, and often reflect biases in their training data. A core issue is their lack of grounding: They cannot reliably connect their outputs to real-world facts. As a result, they may assert incorrect information with apparent certainty, such as occasionally claiming the Earth is flat.
Autonomy in Action: How LLMs Push Agent Capabilities Forward
But despite all these imperfections, LLMs remain potent tools. We asked GPT-4 a logic teaser question, which gave us the correct answer out of the gate, something smaller LLMs struggle with badly, and that no handwritten code could deal with on its own without knowing the question in advance.A recent report from Andreessen Horowitz on emerging LLM stacks sees Agents as purely autonomous software. That means they can plan and make decisions independently of human intervention.
Redefining Agents: Beyond the Myth of the Self-Contained Intelligence
At the AI Infrastructure Alliance (AIIA), we define agents differently. We see them as semi-autonomous software with humans making some of the decisions, aka humans in the loop, and fully autonomous systems. People must understand that an Agent is not usually a singular, self-contained software like the LLM.
We hear the word Agent and it calls to mind a self-contained entity, mostly because we anthropomorphize them and think of them as human, since people are the only benchmark we have for actual intelligence.
Agents as Systems: Orchestrating Models, Tools, and APIs
Agents are usually a system of interconnected software pieces. The HuggingGPT paper from a Microsoft Research team outlines a common and practical approach to modern agents. An LLM uses other models, like an image diffuser, such as Stable Diffusion XL, or a coding model, like WizardCoder, to do more advanced tasks. It may also use APIs in how we use our hands and legs.
It uses those tools as an extension to control outside software or interact with the world. An LLM might train on its API knowledge as part of its dataset, a fine-tuned dataset, or use another external model explicitly trained on APIs, like Gorilla.
Centaurs: Bridging the Gap Between Automation and Oversight
At the AIIA, we see Agents and any software system that interacts with the physical or digital world and can make decisions that were usually only in the realm of human cognition in the past.
We call semi-autonomous agents Centaurs. These are intelligent software with a human in the loop. Agents are fully autonomous or almost fully autonomous pieces of software that can plan and make complex decisions without human intervention.
We can think of a Centaur as an “Agent on rails,” a precursor to fully autonomous Agents. Centaurs can accomplish complex tasks if they're well defined with clear guardrails and someone is checking their work or intervening at various steps.
The Spectrum of Autonomy: From Human-in-the-Loop to Full Independence
Fully autonomous agents that can do their jobs without human intervention are a good example of the levels of autonomy in agentic systems. A good example comes from the world of self-driving cars and is beautifully laid out in the book AI 2041 by Kai-Fu Lee and Chen Quifan.
Autonomous systems are classified by the Society of Automotive Engineers as Level 0 (L0) to Level 5 (L5):
- L0 (zero automation): means the person does all the driving, but the AI watches the road and alerts the driver to potential problems, such as following another car too closely.
- L1 (hands-on): means the AI can do a specific task, like steering, as long as the driver is paying close attention.
- L2 (hands-off): Here, the AI can perform multiple tasks like braking, steering, accelerating, and turning, but the system still expects the human to supervise and take over when needed.
- L3 (eyes off): is when an AI can take over all aspects of driving, but still needs the human to be ready to take over if something goes wrong or the AI makes a mistake.
- L4 (mind off): is where the AI can take over driving altogether for an entire trip, but only on well-defined roads and in well-developed environments that the AI understands very well, like highways and city streets that have been extensively mapped and surveyed in high definition
- L5 (steering wheel optional): means that no human is required at all for any road or environment, and you don't need to have a way for humans to take over, hence the “steering wheel” optional. L0 to L3 is an extra option on a new car, like air conditioning, leather seats, or cruise control. They still need humans at the wheel. These Centaurs need humans in the loop, like most Agents today. Most people would be reluctant to let an Agent compose an email to their boss or mother without reading it before sending it.
Digital L5: The Rise of Fully Autonomous Software Agents
By Level 4 autonomy, the intelligence behind a self-driving vehicle begins to resemble accurate independent decision-making, capable of navigating defined routes without human input. Such systems could power public buses or shuttles operating on fixed paths. At Level 5, vehicles become fully autonomous, able to handle any road or condition without human oversight, enabling around-the-clock deliveries or on-demand robotaxis.
Since the advent of GPT-3 and GPT-4, developers have begun building digital analogs of these L5 systems, fully autonomous software agents. Early experiments like BabyAGI and AutoGPT aim to leverage large language models to complete complex, multi-step tasks independently:
- Planning software projects
- Booking travel
- Curating gifts
- Drafting hiring strategies
These efforts signal the early stages of truly autonomous digital intelligence.
The Roadblocks to Autonomy, and the Engineers Breaking Through
Today’s autonomous agents still struggle with:
- Long-term planning
- Complex reasoning
- Executing end-to-end tasks
While we envision systems capable of designing full marketing campaigns, such capabilities remain out of reach:
- Building websites
- Generating outreach content
- Identifying leads
- Launching communications
Progress is accelerating. As traditional software engineers enter the machine learning space, they bring fresh perspectives and unconventional solutions that complement the strengths of data scientists and ML practitioners.
This influx of new thinking is driving steady improvement. Fully autonomous agents may not yet be ready for prime time, but their emergence as everyday tools, personally and professionally, feels increasingly inevitable within the next decade.
Hype vs. Reality: The Illusion of Progress in Open Source AI
Fully autonomous agent projects have captured widespread public attention, with tools like AutoGPT amassing GitHub stars at record-breaking speed. GitHub stars are no longer a reliable proxy for technical maturity or sustained developer engagement. Much of the enthusiasm surrounding AI is fueled by science fiction and cinematic portrayals, rather than the current realities of the technology.
This mismatch between expectation and execution often leads to disillusionment. Projects may soar in popularity based on the promise of super-intelligent systems, reminiscent of Hollywood’s AI avatars, only to see developer interest fade when the software falls short of those imagined capabilities.
Evolving Intelligence: Memory, Reflection, and the Future of Agent Reasoning
Despite early setbacks, some autonomous agent projects, like BabyAGI, continue attracting dedicated contributors who steadily enhance their capabilities. At the same time, the field of agent reasoning and planning is evolving through new techniques that push the boundaries of what LLMs can do.
Approaches such as Chain of Thought prompting help models reason more effectively by encouraging step-by-step problem-solving. Research efforts like Stanford’s Generative Simulacra explore how agents can develop memory and self-reflection. These systems mark a significant step toward more adaptive and cognitively rich agents by storing natural language records of their experiences, synthesizing them into high-level insights, and retrieving them to guide future behavior.
Limits of Autonomy, and the Shockwave of ChatGPT
Despite all these techniques, they still struggle with going off the rails, hallucinating, and making major mistakes in their thinking, especially as the time horizon for independent decision-making increases. Short-term, on-the-spot reasoning is often sound, but the longer the agents have to act and make decisions independently, the greater their chance of breaking down.
Even with all these limitations and caveats, Agents have become more powerful. Why have agents suddenly become much more powerful? The answer is simple. ChatGPT was a watershed computing and AI history that shocked outsiders and insiders alike.
From ELIZA to ChatGPT: The Evolution of Conversational Intelligence
Suddenly, we had a system that delivered realistic and free-flowing conversations on any subject. That's a radical departure from the past, where chatbots were brittle and not even vaguely human. The first chatbot, ELIZA, was created at MIT in the 1960s.
We've had Clippy, the famous paperclip in Microsoft Office products in the late 90s and early 2000s, notorious for being slow and virtually useless for answering questions. We’ve had Alexa and Siri, which can play songs or answer questions by doing database lookups. But none of them worked all that well.
ChatGPT and GPT-4 just feel different.
That's because all these bots of the past were often brittle, rule-based systems. They were glorified scripts that triggered based on what you said or wrote. They couldn't adapt to you or your tone or writing style. They had no real context about your larger conversation with them. They felt static and unintelligent. Nobody would be able to mistake them for humans.
GPT-4 and the Mystery of Scale: The Hidden Architecture Behind the Benchmark
The architecture of GPT-4 remains undisclosed, though it's known to be based on the transformer model. Speculation abounds, with some suggesting it’s a single massive transformer with a trillion parameters.
In contrast, others propose it uses a Mixture of Experts (MoE) approach, leveraging multiple smaller expert models for specialized tasks. Despite its actual structure, GPT-4 remains the most potent and capable AI model on the market, setting the benchmark in the field. Even with open-source models like Meta’s LLaMA 2, released a year later, approaching its performance, they have yet to surpass GPT-4’s capabilities.
That said, it's only time before other teams create a more powerful model. By the time you read this, the arms race to create ever more powerful models by open-source teams like Eleuther AI and Meta’s AI research division, or by any of the proprietary companies piling up GPUs to build their own, may already have produced that model, like:
- Anthropic
- Cohere
- Inflection
- Aleph Alpha
- Mistral
- Adept
Beyond RPA: The Rise of Adaptive, AI-Driven Agents
Today's more powerful AI-driven agents, fueled by advanced software, enable significantly enhanced capabilities. Unlike the limited, process-driven enterprise Robotic Process Automation (RPA) systems of the past, designed primarily for structured data and well-defined tasks, modern agents can operate effectively in the unstructured domains of:
- Websites
- Documents
- APIs
These agents can summarize content, understand text, offer insights, and perform roles such as language tutoring and research assistance, among many other applications.
The Open-Source Explosion: Competing Models and the Future of AI Innovation
The launch of ChatGPT marked the beginning of a new era, but it is far from the end. Since then, there has been a surge in powerful open-source models, with platforms like Hugging Face tracking their performance through a public leaderboard. Models have risen to prominence, like:
- Meta’s LLaMA
- Llama 2
- Vicuna
- Orca
- Falcon
- Specialized models like Gorilla
Venture capital is flooding into foundation model companies, enabling the creation of large-scale GPU supercomputing infrastructures. OpenAI has secured over $10 billion in investments, and recently, Inflection AI raised $1.3 billion to build a 22,000 Nvidia H100 GPU cluster for training advanced models. With such significant backing, OpenAI will face growing competition, and at AIIA, we anticipate a surge of new, capable models that will drive the intelligence applications of the future.
The Rise of Lightweight, AI-Powered Development Teams: Leveraging LLMs for Scalable Software
Agents represent a new generation of software, surpassing the capabilities of traditional hand-coded applications. With the power of large language models (LLMs) and the expanding middleware ecosystem, even small teams, ranging from one to ten developers, can now create competent AI-driven applications.
This shift mirrors the “WhatsApp effect,” where a small team of 50 developers reached 300 million users by leveraging an increasingly sophisticated stack of pre-built software tools, from user interfaces to secure encryption libraries. This new landscape enables faster, more scalable development and innovation by reducing the need for extensive in-house coding.
The Democratization of Software Development: LLMs and Specialized Models Empowering Small Teams
The combination of powerful large language models (LLMs) and specialized task-specific models alongside a new generation of middleware has significantly lowered the barriers to building sophisticated software, such as:
- SAM (Segment Anything Model)
- Stable Diffusion
- Gen1
- Gen 2
As a result, even small teams can now reach broader audiences and develop impactful applications.
This shift has created more focused, intelligent applications, such as bots capable of analyzing legal documents or researching potential marketing leads. By stacking these agents together, we can make intelligent microservices that provide innovative functionality, marking a new era of software development with rapid innovation and a flurry of new applications.
The Emergence of AI-Powered Agents: From Sci-Fi Concepts to Real-World Applications
Advances in large language models (LLMs) have unlocked the potential for agents capable of performing tasks once confined to science fiction. These agents can now process sensory inputs from:
- Keystrokes
- Web pages
- Code
- External knowledge repositories
This enables them to accomplish complex tasks like:
- Automatically enhancing photos
- Analyzing web pages or PDFs
- Making intricate decisions
The once-fantastical trope of “enhancing” grainy footage, commonly seen in detective films, has become a reality with AI systems that can unveil hidden details. The landscape of agent development has evolved from being exclusive to robotics researchers and data scientists to including traditional programmers, who now leverage these AI-powered agents to accomplish tasks previously deemed impossible with conventional methods.
The Promises and Perils of LLMs and Generative AI: Challenges in Creating Reliable Software
While the capabilities of LLMs and generative AI models are transformative, they come with inherent challenges. Unlike traditional hand-coded software, which fails in predictable ways, LLMs are non-deterministic, leading to wildly unpredictable results. For example, a diffusion model like Stable Diffusion XL may perform excellently at photorealistic portraits but struggle with a cartoon-style image.
The open-ended nature of these models means that testing for every potential use case is virtually impossible. Users may interact with these systems in vastly different ways, ranging from simple inquiries to attempts at exploiting vulnerabilities. As a result, harnessing these systems to create dependable software remains a significant challenge. This subtopic explores the opportunities and risks presented by LLMs, generative AI, and agents for businesses of all sizes.
The Three Approaches to AI/ML Platforms
There are three primary approaches to building an AI/ML platform:
- Build your own
- Buy an end-to-end solution
- Best of breed
When building a web application platform or in-house IT system, organizations face many of the same considerations as in AI/ML development, though with some key differences. The most significant distinction is the relative immaturity of the enterprise AI/ML field, which results in a broad array of products and services. In some areas, clear market leaders have emerged, while in others, numerous options may align with specific organizational needs.
This diversity mirrors the early stages of other technological advancements, such as the automobile industry, where countless small car manufacturers existed before Ford's assembly-line model dominated, or the early days of the web, which saw a wide variety of web servers before a few key players, like Apache and NGINX, emerged as market leaders.
Build Your Own
Initially, tech companies like Google, Tesla, OpenAI, DeepMind, and Netflix were the first to scale AI/ML techniques, often building their solutions for developing and deploying models. However, as AI/ML techniques matured and demand grew, enterprise software companies and startups emerged to offer solutions for organizations lacking the in-house expertise or resources to build and maintain custom systems. The AIIA advises against most companies attempting to develop their AI/ML platform from scratch or relying solely on open-source components.
Such an approach is highly complex and likely to fail, suited only for advanced teams with particular needs. Instead, organizations should consider leveraging existing, developed tools. According to our enterprise survey, only 20% of companies build their entire infrastructure in-house, 45% use a mix of in-house and third-party tools, and 31% rely solely on third-party solutions.
The Challenges of Building vs. Buying AI/ML Infrastructure
As AI/ML infrastructure matures, many companies are moving away from custom-built solutions in favor of a hybrid approach or purely purchasing pre-built platforms. Custom-built applications often require substantial engineering resources for long-term maintenance, and teams frequently discover that their platform, initially designed for specific use cases, becomes too rigid and brittle to support broader needs.
The third-largest challenge faced by teams when building their AI/ML infrastructure was realizing that their platform was suitable only for specific applications, limiting its scalability and flexibility.
The Evolution and Limitations of Custom AI/ML Platforms
The Michelangelo platform at Uber, led by Mike Del Blaso, is a key example of the limitations of custom-built AI/ML infrastructure. While it was highly effective for specific use cases like UberEats, it struggled with generalizability to broader applications. This led Uber to transition to newer platforms and fragment Michelangelo's components into smaller, more flexible open-source projects.
Despite the rise of robust software platforms that address most AI/ML lifecycle needs, advanced teams are still expected to build some bespoke components, such as:
- Custom workflows
- Glue code
- In-house libraries
This trend is anticipated to persist over the next five years as platforms evolve to meet increasingly complex needs.
The Risks of Adopting a Single, Unified AI/ML Platform
While purchasing a single, unified AI/ML platform to address all machine learning and analytics needs seems appealing, the AIIA advises against this approach. Drawing parallels to past industry trends, such as the widespread adoption of Oracle databases and VMware suites, this strategy may not be ideal for AI/ML infrastructure, which is still in its early adopter phase.
As sociologist Everett Rogers outlined in Diffusion of Innovation and Geoffrey Moore expanded in Crossing the Chasm, adopting new technology is often uneven, with organizations at different stages of readiness. A unified solution may not be flexible enough to meet evolving needs in the fast-developing AI landscape.
The Evolving Landscape of AI/ML Platforms: A Multi-Solution Approach
At this stage of technological development, no single AI/ML platform has emerged as the dominant, all-encompassing solution. Instead, various rapidly evolving platforms address different aspects of:
- Model development
- Training
- Deployment
- Management
Over time, these platforms are expected to expand their:
- Capabilities
- Consolidate
- Merge
Comprehensive and robust platforms typically solidify during the late stages of a technology’s lifecycle, from the early majority to the late majority phases, underscoring the dynamic and fragmented nature of the current AI/ML ecosystem.
Scrutinizing Vendor Claims in the AI/ML Platform Market
Despite the marketing claims of some vendors asserting that their solution can cover every aspect of the AI/ML lifecycle, organizations should remain cautious. No solution currently addresses the full breadth of innovation across key areas such as:
- Data ingestion
- Versioning
- Synthetic data generation
- Feature stores
- Model registries
- Orchestration systems
- Deployment
- Monitoring
When evaluating AI/ML platforms, organizations must critically assess vendor capabilities and inquire thoroughly about their ability to support the diverse and evolving needs of the AI/ML ecosystem.
Building an AI/ML Platform Around Core Vendors
Organizations can strategically choose one or two vendors as the foundation of their AI/ML platforms and then build around those core solutions. Many platforms offer broad capabilities suitable for complex enterprises, but organizations must understand their current and future needs before deciding.
For example, focusing on structured and semi-structured data for analytics tasks might make a platform like Spark ideal today. Still, future needs, such as deep learning for video analytics, could reveal its limitations. While a core vendor may provide essential features, specialized vendors may be needed to fill in:
- Monitoring
- Observability
- Explainability gaps
The Challenges of Cloud Vendor AI/ML Solutions
While cloud vendors offer seemingly end-to-end AI/ML solutions, a closer look often reveals significant gaps. For instance, Amazon’s SageMaker suite, which includes tools for data wrangling and pipelines, comprises standalone tools that may lack seamless integration.
These tools are often tailored for structured data use cases and struggle with handling unstructured data, such as:
- Video
- Images
- Free-form text
Furthermore, many cloud vendors rely on internally developed tools instead of adopting well-established, industry-leading platforms. This reflects the early stage of the AI/ML adoption curve, where no clear market leader has emerged.
Evaluating Cloud Vendor AI/ML Solutions in the Early Adoption Phase
As AI/ML tools become more widespread, cloud vendors will likely replace parts of their suite with more widely adopted, best-of-breed alternatives. This raises the question of why not start with the most established solutions from the outset. For example, Amazon’s feature store may not become the long-term standard compared to dedicated open-source solutions like Feast or commercial platforms like Tecton, which offer multi-cloud support.
While public clouds excel at commoditizing mature technologies, AI/ML is still in the early adoption phase. Despite marketing claims of comprehensive, end-to-end solutions, evaluating cloud vendor solutions with the same scrutiny as other offerings is essential.
Best-of-Breed Approach for Building an AI/ML Stack
The AIIA recommends a modular, best-of-breed approach for medium to advanced data science and engineering teams when building an AI/ML stack. This strategy involves selecting leading platforms for core capabilities such as:
- Data processing
- Pipelining
- Versioning
- Lineage
- Experiment tracking
- Deployment
Once a robust core platform is chosen, organizations can incorporate specialized satellite platforms to address more specific needs, such as:
- Synthetic data generation
- Feature stores
- Advanced monitoring
- Observability
- Explainability tools
This approach ensures flexibility and scalability while meeting the unique requirements of each use case.
Evaluating and Selecting Core AI/ML Platforms for Long-Term Success
At the current stage of AI/ML evolution, adopting a best-of-breed solution requires thoughtful integration and strategic planning. When selecting core platforms, ensure they feature clean, well-documented APIs and simple ingress and egress points. If these platforms already integrate with others you're considering, evaluate the depth of these integrations to determine if they are loosely or tightly connected at multiple levels.
To choose the right core platform, assess your current machine-learning use cases and those anticipated over the next year and five years. Given the rapid advancements in AI/ML technology and infrastructure, predicting all future use cases is challenging. Therefore, focus on platforms that can accommodate your needs during the expected timeframe, ensuring scalability and flexibility as your business evolves.
Future-Proofing Your AI/ML Platform Selection for Evolving Use Cases
While predicting every future use case is impossible, choosing a platform that allows expansion into unforeseen applications is essential. Look for platforms that offer maximum flexibility, language agnosticism, and the ability to process a variety of data types:
- Structured
- Semi-structured
- Unstructu
This ensures your core platform can support current and future needs as your team moves from initial use cases like churn prediction and customer-demand forecasting to more advanced tasks such as:
- Computer vision
- Audio transcription
- Natural language processing (NLP)
Select a core platform that is adaptable, scalable, and capable of supporting diverse use cases. This will enable your team to evolve without imposing technological constraints.
Evaluating AI/ML Platforms Beyond Marketing Claims
When assessing AI/ML platforms, it's crucial not to rely solely on marketing claims of comprehensive capabilities. Instead, focus on well-documented, real-world use cases and examples that align with your current and anticipated needs. Choosing the wrong core platform can be costly and lead to the need for multiple supplementary platforms to cover unmet requirements
While replacing a monitoring platform is relatively easy, swapping out core systems like pipeline and orchestration platforms is far more complex and disruptive. Thorough evaluation and understanding of the platform’s practical applications and limitations are essential for long-term success.
Challenges of the Best-of-Breed Approach in AI/ML Platforms
While a best-of-breed strategy maximizes success in the evolving AI/ML landscape, it presents challenges, particularly in cost and support. The need to purchase multiple specialized platforms can increase costs, which must be weighed against the expense of developing and maintaining an in-house system or dealing with the limitations of an “end-to-end” solution that fails to deliver.
Support becomes more fragmented, requiring teams to manage multiple contracts and service providers. With modern enterprises accustomed to juggling various support agreements, this challenge is often less daunting than it was in the past. Despite these drawbacks, the benefits of tailored, specialized solutions usually outweigh the complexities.
Steps to Building a Strong AI Infrastructure Ecosystem
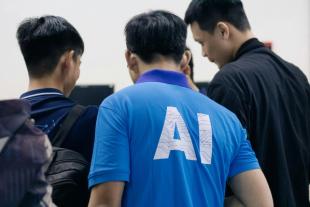
AI applications vary widely across industries, addressing unique challenges and offering competitive advantages specific to each sector. Customizing AI deployments based on industry needs is critical for success. For example, organizations in financial services use AI to optimize fraud detection and automate compliance checks. Healthcare organizations rely on AI for advanced diagnostics and predictive patient care.
Retail and manufacturing use AI for inventory forecasting, automation, and customer personalization. This industry focus enables AI to generate tangible results, making it essential for companies to tailor their AI investments based on their sector’s unique requirements.
Integrate AI Across Business Domains
Beyond industry-specific applications, AI can be deployed in functional areas across the organization, enhancing business processes and customer interactions.
Several key domains where AI can drive value include:
- Human Resources: AI can optimize recruiting, training, and employee engagement, using predictive analytics to identify potential skill gaps or retention risks.
- Customer Service: AI chatbots and automated support systems instantly respond to customer queries, improving response times and customer satisfaction.
- Sales and Marketing: AI-driven insights help teams analyze consumer behavior, forecast sales trends, and personalize marketing campaigns.
By incorporating AI across multiple business functions, organizations can create a cohesive approach to automation and intelligence that enhances operational efficiency and customer engagement.
Develop Robust AI Infrastructure and Techniques
Building an effective AI ecosystem requires a robust infrastructure that supports AI’s data, processing, and integration needs.
An AI-focused tech stack includes:
- Data Management: It is fundamental to ensure that data is accessible, high-quality, and ready for AI processing. A well-structured data management system is critical.
- AI Engineering and Operations: AI engineering integrates AI into business operations. AI operations (AI Ops) support the ongoing management of AI models to ensure their accuracy and reliability over time.
- Machine Learning and Natural Language Processing (NLP): These are core techniques in many AI solutions, powering everything from automated customer responses to predictive maintenance in manufacturing.
Investing in these core areas allows organizations to build and sustain AI models that scale as business needs evolve.
Prioritize Governance and Risk Management
As AI becomes more embedded in organizational processes, managing risks and ensuring ethical AI use is increasingly critical. A governance structure should focus on:
- Transparency and Interpretability: Ensuring that AI decisions can be explained and understood is key, especially in highly regulated industries.
- Ethics and Privacy: Concerns over AI-driven decisions affecting privacy or fairness require organizations to develop frameworks that address ethical considerations and safeguard personal data.
- Risk Management: AI can introduce new risks, such as biases in decision-making or security vulnerabilities.
By implementing strong governance, businesses can mitigate risks and maintain trust. A robust governance model can prevent pitfalls and ensure that AI-driven initiatives align with regulatory standards and ethical practices.
Stay Ahead of Key Trends and Emerging AI Applications
AI is rapidly evolving, and staying informed of emerging trends is essential for future-proofing AI strategies. Business leaders must monitor advancements in areas like:
- Generative AI: Tools like language models and AI-generated content offer companies new ways to automate content creation and customer interactions.
- Quantum Computing: Though still emerging, quantum computing has the potential to solve complex problems that traditional computers cannot address, which could revolutionize AI applications.
- Employee Augmentation: AI is increasingly used to augment human tasks, allowing employees to focus on strategic activities while AI handles repetitive tasks.
By monitoring these trends, companies can position themselves to adopt new AI advancements and maintain a competitive edge.
Practical Steps to Implement an AI Framework
Establishing a thriving AI ecosystem requires thoughtful planning and execution.
Here are actionable steps based on recommendations:
- Begin with Pilot Projects: Start with smaller-scale AI projects in high-impact areas to demonstrate value and refine processes. Successful pilots can build internal support and inform larger deployments.
- Invest in Data Infrastructure: Prioritize data quality and accessibility. Strong data foundations are crucial for AI reliability.
- Set Up AI Governance Early: Define an AI governance structure to address risks, ensure compliance, and maintain ethical standards.
- Prioritize Continuous Learning: As AI technology advances, upskill teams and invest in training to keep your workforce AI-ready and informed about the latest techniques and tools.
Building an effective AI ecosystem is more than just deploying technology—it requires a strategic approach that aligns AI initiatives with specific business goals and industry demands. Following this framework, business leaders can harness AI to drive meaningful value and build a resilient, future-ready organization.
Start Building with $10 in Free API Credits Today!
Inference delivers OpenAI-compatible serverless inference APIs for top open-source LLM models, offering developers the highest performance at the lowest cost in the market. Beyond standard inference, Inference provides specialized batch processing for large-scale async AI workloads and document extraction capabilities designed explicitly for RAG applications.
Start building with $10 in free API credits and experience state-of-the-art language models that balance cost-efficiency with high performance.