The Ultimate Guide to LLM Inference Optimization for Scalable AI
Published on Mar 13, 2025
Large language models have quickly become essential tools for various AI applications. With their incredible performance comes a significant cost—especially during inference. For instance, a single query to an advanced model like OpenAI's GPT-3 can cost over $0.03. When deployed at scale, the cost of querying these models can become prohibitive, and slow response times can hamper user experience. LLM inference optimization solves these challenges and can help businesses achieve lightning-fast, cost-efficient, and scalable AI inference without sacrificing model accuracy or performance. Additionally, understanding AI Inference vs Training is crucial to optimizing model performance and ensuring smooth deployment.
AI inference APIs can improve LLM inference speed and reduce costs. These tools can help businesses integrate their optimization processes seamlessly and achieve their objectives faster without building optimization solutions from scratch.
What Is LLM Inference Optimization?
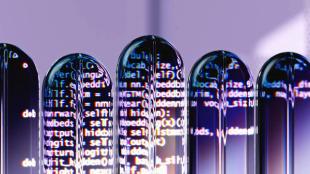
LLM inference optimization is a way to make large language models (LLMs) run more efficiently. When LLMs are used for inference, they can be slow and resource-intensive, especially for larger models with billions of parameters. This can cause latency issues for applications using LLMs to generate real-time responses. Inference optimization aims to improve LLMs’ speed, efficiency, and resource utilization without compromising performance.
This is crucial for deploying LLMs in real-world applications where low latency and high throughput are essential for a seamless user experience. For example, retrieval-augmented generation (RAG) pipelines, which incorporate external information into LLM prompts, can significantly increase the processing workload. Optimizing inference becomes even more critical in such scenarios to ensure timely and efficient responses.
Optimization Techniques
Several techniques are employed to optimize LLM inference. These can be broadly categorized into:
1. Quantization Optimizes LLM Inference
Quantization reduces the numerical precision of model parameters, such as weights and activations. This technique minimizes the number of bits needed to represent signals and data within the model, leading to a smaller model size and faster processing.
This, in turn, reduces the model’s memory footprint and computational requirements, leading to speedier inference and lower memory usage.
How is it Done?
Quantization techniques map floating-point numbers, typically 32-bit, to lower-precision representations like 8-bit or even 4-bit integers. This can be done post-training (PTQ) or during training (QAT). PTQ involves converting the model’s weights after training, while QAT integrates quantization into the training process.
One standard method is the affine quantization scheme, which involves scaling and shifting the original values to fit the lower precision range. This scheme helps to minimize information loss during the conversion process.
Benefits
- Quantization significantly reduces the model’s size, enabling deployment on devices with limited memory.
- Lower precision arithmetic leads to faster computations, improving inference speed.
- Smaller models are easier to scale, efficiently handling larger workloads.
- Quantized models require less energy, making them more cost-effective and environmentally friendly.
Limitations
- Quantization can reduce model accuracy due to the approximation error introduced by lower precision.
- Significant activation outliers in LLMs can pose challenges for quantization, potentially degrading performance.
Open research problems:
Research is ongoing to develop quantization techniques that minimize the impact on model accuracy. Finding effective ways to handle outliers during quantization is an active research area. More comprehensive evaluation frameworks are needed to assess the performance of quantized LLMs across diverse tasks.
2. Knowledge Distillation Boosts LLM Inference
Knowledge distillation transfers knowledge from a larger, more complex LLM (teacher) to a smaller LLM (student). This allows the student model to achieve comparable performance with a smaller size and reduced computational requirements.
How is it done?
The teacher model is first trained on a large dataset. Then, the student model is trained using the teacher’s output probabilities (soft targets) and the ground truth labels (challenging targets). This helps the student model learn the underlying knowledge and patterns captured by the teacher.
Benefits
- Distillation creates smaller models that are easier to deploy and require less computational resources.
- Smaller models lead to faster inference, making them suitable for real-time applications.
- Due to the knowledge transfer process, student models can sometimes generalize better than the teacher model.
Limitations
- The student model may not achieve the same level of accuracy as the teacher model.
- Distillation relies on the availability of a well-trained teacher model.
Open research problems: Research focuses on enhancing distilled models’ generalization ability across different tasks and domains. Efficiently scaling distillation for larger models and datasets is an ongoing challenge. Developing techniques for knowledge distillation across different tokenizers is an active research area.
3. Architectural Optimization Improves LLM Inference
Architectural optimization involves modifying the structure and design of LLMs to improve inference efficiency.
This can include techniques like:
- Layer reduction: Reducing the number of layers in the model can lead to smaller models and faster inference.
- Attention mechanism optimization: Employing efficient attention mechanisms like FlashAttention can reduce computational complexity. Another technique is paged attention, which improves memory management for large models and long input sequences using paging techniques similar to those used in operating system virtual memory. This effectively reduces fragmentation and duplication in the key-value cache, allowing for longer input sequences without running out of GPU memory.
Common attention optimisation techniques:
- Parameter sharing: Sharing parameters across different model parts can reduce the overall number of parameters.
- Parameter-Efficient Fine-Tuning (PEFT): PEFT techniques aim to adapt LLMs to specific tasks by fine-tuning only a tiny subset of the model’s parameters, reducing memory requirements and potentially improving inference efficiency.
Exploring Low-Rank Adaptation (LoRA): How It Enhances Efficiency in LLMs
Below is a description of a PEFT technique called Low-Rank Adaptation (LORA).
How is it done?
Architectural optimization often involves experimenting with different model configurations and training strategies to find the most efficient design for a given task. This can include modifying the number of layers, attention heads, hidden units, and other architectural parameters.
Benefits
Optimized architectures can lead to faster inference and reduced computational costs. Architectural modifications can result in smaller models with lower memory requirements. Optimized architectures can sometimes lead to improved accuracy and performance on specific tasks.
Limitations Architectural optimization can be complex and require significant expertise. Optimized architectures may be specific to certain tasks and not generalize well to others.
Open research problems
Research is ongoing to design new LLM architectures that are inherently more efficient for inference. New techniques for optimizing LLM architectures are constantly being explored. Research is focused on overcoming the limitations of current LLM architectures, such as token limitations and hallucinations.
4. Memory Optimization Techniques
In addition to the core optimization techniques discussed above, memory optimization is crucial in efficient LLM inference.
Two prominent techniques in this area are:
- KV Cache Compression: The key-value (KV) cache in transformer-based LLMs stores past activations to improve efficiency. It can become a significant memory bottleneck. KV cache compression techniques aim to reduce the memory footprint of the KV cache, allowing for longer input sequences and more efficient inference. One common approach is KV cache quantization, where the data in the KV cache is stored with lower precision.
- Context Caching: Context caching involves storing the intermediate representations of previously processed inputs. When a similar input is encountered, the cached representation can be reused, avoiding redundant computations and speeding up inference. Google has recently released context caching features in its LLM serving frameworks, highlighting the growing importance of this technique.
5. Pruning LLMs to Improve Inference
Pruning eliminates neurons, connections, and unimportant weights in the model that do not significantly contribute to performance. The model becomes smaller and faster while retaining its performance edge.
- Structured pruning removes more significant parts of the network, such as neurons. This can change the model network.
- Unstructured pruning eliminates parameter weights so the model network does not change.
6. Dynamic Batching for Cost-Effective LLM Inference
Dynamic batching combines multiple text generation requests into a single batch. Instead of handling individual requests independently, they are processed as a batch by the efficient parallel processing capabilities of GPUs or TPUs.
This means increased throughput, improved resource utilization, and cost efficiency. This technique is ideal for situations where large volumes of text generation are needed.
7. Parallelism Improves LLM Inference Efficiency
Dynamic batching combines multiple text generation requests into a single batch. Instead of handling individual requests independently, they are processed as a batch by the efficient parallel processing capabilities of GPUs or TPUs.
This means increased throughput, improved resource utilization, and cost efficiency. This technique is ideal for situations where large volumes of text generation are needed.
Tensor Parallelism
Instead of a single processing unit handling the entire model, it is distributed across multiple processing units. This speeds up inference and is helpful for large models. It also results in better resource utilization and improved scalability.
Pipeline Parallelism
It distributes the various layers of a model (including inference) across multiple devices. You don't need to invest in or deploy expensive high-memory devices to run large models. For example, a retail chain running LLMs across thousands of stores might use pipeline parallelism to run their large pricing models on less expensive hardware spread across stores.
8. FlashAttention for Efficient LLM Inference
FlashAttention is an optimized version of the computationally intensive attention mechanism used in transformer models, commonly found in LLMs. It enhances memory access patterns and removes redundant calculations from the attention process.
This results in faster inference times and reduced memory usage, delivering considerable savings in large-scale deployments.
9. Edge Model Compression for LLMs
Edge model compression reduces large models to run on edge devices like smartphones, IoT devices, or other hardware with limited computing power. Techniques like quantization, weight sharing, and low-rank approximation ensure model performance in the new environment.
Balancing Speed and Accuracy: Understanding the Trade-Offs in Inference Optimization
While inference optimization is a practical necessity, it is essential to remember that these techniques come with accuracy trade-offs and may incorporate user subjectivity into the model during optimization.
Related Reading
- Model Inference
- AI Learning Models
- MLOps Best Practices
- MLOps Architecture
- Machine Learning Best Practices
- AI Infrastructure Ecosystem
4 Key LLM Inference Optimization Benefits
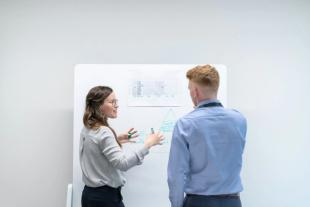
1. Slash Operational Costs with LLM Inference Optimization
Companies leveraging large language models gain significantly from LLM inference optimization. Let’s look at some of them—reduced operational costs. The main reason behind the high costs of LLMs is their substantial computational resources.
Inference optimization reduces the following:
- Cloud costs: Typically, cloud providers charge by usage. Inference optimization drives down this spend by reducing the length of compute instances.
- Energy consumption: Inference optimization reduces energy needs, making the LLM more eco-friendly
- Infrastructure optimization: It allows for efficient use of the available hardware.
2. Boost Response Times
Imagine a customer or stakeholder waiting several minutes after submitting a complex query on one of your digital channels. They will find this irritating and even distressing, especially if the concern concerns critical matters like medical care or disaster response. Optimizing inference lowers latency and ensures the model serves faster, significantly improving response times and eliminating user friction.
3. Seamlessly Scale Applications
Applications that have to scale user bases rapidly for profitability, such as an ecommerce platform or a social media app, will be constrained by non-streamlined inference. Inference optimization, which reduces LLM’s resources, enables the model to handle innumerable users simultaneously, enabling scalability.
4. Improve Personalization for Better User Experiences
The modern customer wants speed and personalization. Techniques like knowledge distillation and pipeline parallelism help LLMs deliver these more contextualized, customized interactions at the same speed, if not faster than non-optimized models, vastly enhancing user satisfaction.
How Serverless Inference is Transforming AI Deployment for Developers
Inference delivers OpenAI-compatible serverless inference APIs for top open-source LLM models, offering developers the highest performance at the lowest cost in the market.
Beyond standard inference, Inference provides specialized batch processing for large-scale async AI workloads and document extraction capabilities designed explicitly for RAG applications.
Start building with $10 in free API credits and experience state-of-the-art language models that balance cost-efficiency with high performance.
Related Reading
- AI Infrastructure
- MLOps Tools
- AI as a Service
- Machine Learning Inference
- Artificial Intelligence Cost Estimation
- AutoML Companies
- Edge Inference
LLM Inference Optimization Platforms and Tools
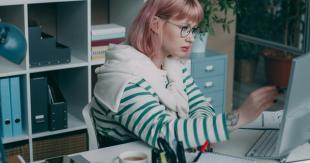
Optimizing large language model inference requires the proper hardware. GPUs are the best option for many tasks, with their many cores, high memory bandwidth, and support for the tensor cores that accelerate mixed-precision calculations.
While NVIDIA GPUs dominate this space, others from AMD and Intel can perform well for large language models. TPUs, or tensor processing units, also excel at LLM inference and are particularly well-suited for models built using Google’s TensorFlow framework. FPGAs, or field-programmable gate arrays, can be customized for specific tasks, making them an excellent choice for LLM inference for organizations with specialized needs.
Software Platforms: Streamline Your LLM Inference Tasks.
Various software platforms can help with LLM inference optimization. For example, Hugging Face and NeMo offer tools and libraries for optimizing models built using their frameworks, while TensorFlow and PyTorch include modules for improving large model performance.
Popular model deployment platforms like Triton Inference Server and Seldon offer built-in capabilities for optimizing LLM inference.
Tools and Libraries: The Secret Weapons of LLM Inference Optimization
A variety of tools and libraries help with LLM inference optimization, including methods for:
- Quantization
- Pruning
- Distillation
- Transfer learning
For instance, Hugging Face offers robust libraries for model quantization and pruning. Both TensorFlow and PyTorch also have built-in capabilities for these techniques. Hugging Face and Google’s DeepMind have released libraries specifically for model distillation. Transfer learning is a built-in capability of LLMs that allows users to fine-tune pre-trained models on specific tasks.
Choosing the Right Optimization Technique
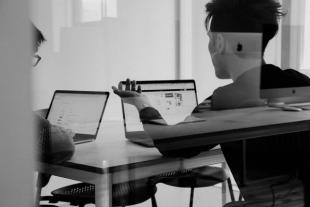
While optimization techniques can enhance model performance, selecting the right approach depends on your objectives and requirements.
- Aiming for improved speed? Quantization can help, but you may need to sacrifice some accuracy.
- Looking also to maintain accuracy?
Knowledge distillation can also help here, but it requires a lot of resources and time to implement.
Want to explore architectural optimization? This technique offers the potential for substantial efficiency gains, but it can get complex and requires specialized expertise. The choice of technique depends on factors such as:
- The desired performance level
- Available resources
- Deployment environment
- Specific application requirements
Careful consideration of these factors is crucial for selecting the most effective optimization strategy.
Quantization: Speed vs. Accuracy
Quantization reduces the precision of the numbers used to represent model parameters. For example, instead of using 32-bit floating-point numbers, quantization might allow the model to use 8-bit integers instead. This process can compress model size by anywhere from 50% to 90%, enabling faster loading times and less memory usage.
Quantization can also lead to degraded accuracy. This loss occurs because lower-precision numbers have less representational capacity than their higher-precision counterparts. In many cases, quantization can be performed with minimal accuracy loss, but it’s essential to carefully evaluate the effects on your specific model before and after implementation.
Knowledge Distillation: Transfer Learning for Smaller Models
Knowledge distillation enables smaller neural networks to learn from more extensive, pre-trained models.
- A teacher model (the more comprehensive, pre-trained neural network) can predict training data.
- A student model (the smaller neural network) is trained to replicate the teacher’s predictions instead of the ground truth.
Knowledge Distillation in AI: How Smaller Models Retain Performance Efficiency
This process allows the smaller model to capture more of the teacher’s knowledge, leading to improved performance on downstream tasks, even when the student model is less accurate than the teacher.
Inference optimization via knowledge distillation can help reduce model size and improve speed while maintaining task performance. Distillation can also be resource-intensive and should be evaluated carefully to ensure it meets optimization goals.
Architectural Optimization: Customizing Your Model
Architectural optimization techniques allow developers to tailor neural network structures to specific tasks and performance goals. By adjusting the number of layers, nodes, and connections, architectural optimization can reduce model size and improve inference speed without significantly losing accuracy.
These techniques can improve downstream task performance in some cases. Architectural optimization can be applied to any neural network, including transformer models for natural language processing tasks. However, these techniques can be complex and require substantial expertise to implement.
Start Building with $10 in Free API Credits Today!
Inference delivers out-of-the-box OpenAI-compatible serverless inference APIs for top open-source LLM models, offering developers the highest performance at the lowest cost.
Beyond standard inference, Inference provides specialized batch processing for large-scale async AI workloads and document extraction capabilities designed explicitly for RAG applications.
Start building with $10 in free API credits and experience state-of-the-art language models that balance cost-efficiency with high performance.
Related Reading
- LLM Serving
- LLM Platforms
- Inference Cost
- Machine Learning at Scale
- TensorRT
- SageMaker Inference
- SageMaker Inference Pricing